First-year students Ming-Hsuan Wu and Chia-Ming Lee from the Institute of Data Science at NCKU won the Special Jury Award at IEEE ICCV 2023. They attended the competition in Paris in September, where they had to develop a system with stable performance using a limited training dataset. Their task involved predicting the positions of players and the ball on a basketball court. By analyzing the boundaries of the basketball court and the distinctive features of players, they transformed the data and expanded it based on known information, achieving remarkable results with minimal computing resources.
Assistant Professor Chih-Chung Hsu explained that IEEE ICCV is the top conference in the field of computer vision globally, attracting over 7,000 participants in recent years. The competition didn't allow pre-training models, making it more challenging to compete against top experts in the field. The team spent a significant amount of time developing an efficient method for memory access, using only one display card for training. Their innovative approach challenged the conventional thinking that large models and extensive GPU usage were necessary for good results, providing a valuable and creative solution. In addition to achieving a top-ranking performance, they received the Special Jury Award from the judges.
In another competition, students Yu-Chung Chiang, Wen-Hai Tseng, and Wei-Hao Huang from the Institute of Data Science at NCKU secured the third position globally at the "ICME2023 Grand Challenge – Low-power Deep Learning Object Detection and Semantic Segmentation Multitask Model Compression Competition for Traffic Scene in Asian Countries." The competition, sponsored by MediaTek, focused on developing a universal model to simultaneously perform semantic segmentation and object detection on a low-power mobile platform, specifically targeting Asian cities with many motorcycles. Their innovative solutions and practical skills helped them stand out among the 223 participating teams.
Furthermore, research assistant Chi-Han Tsai from ACVlab won the world championship for the second consecutive year at the COVID-19 Detection Challenge in May. By analyzing key features learned by AI models, he modified the model's architecture to automatically remove irrelevant and unimportant regions from CT images, producing better detection results. With an accuracy rate of nearly 90%, Tsai achieved the world's first place in identifying lung slices from over 500 sets of data.
Assistant Professor Chih-Chung Hsu expressed his commitment to nurturing talents at the university. He emphasized the importance of encouraging students to participate in international competitions, allowing them to showcase their cross-disciplinary learning outcomes. He mentioned that compared to domestic competitions, international competitions provide a platform for students to be recognized globally. He encourages students to participate in these competitions to enhance their learning and problem-solving skills.
The achievements of students at the NCKU in various international competitions demonstrate their excellence in data science, image recognition, and related fields. The university aims to continue fostering a conducive environment for students to participate in such competitions, providing them with opportunities to showcase their skills on the global stage.
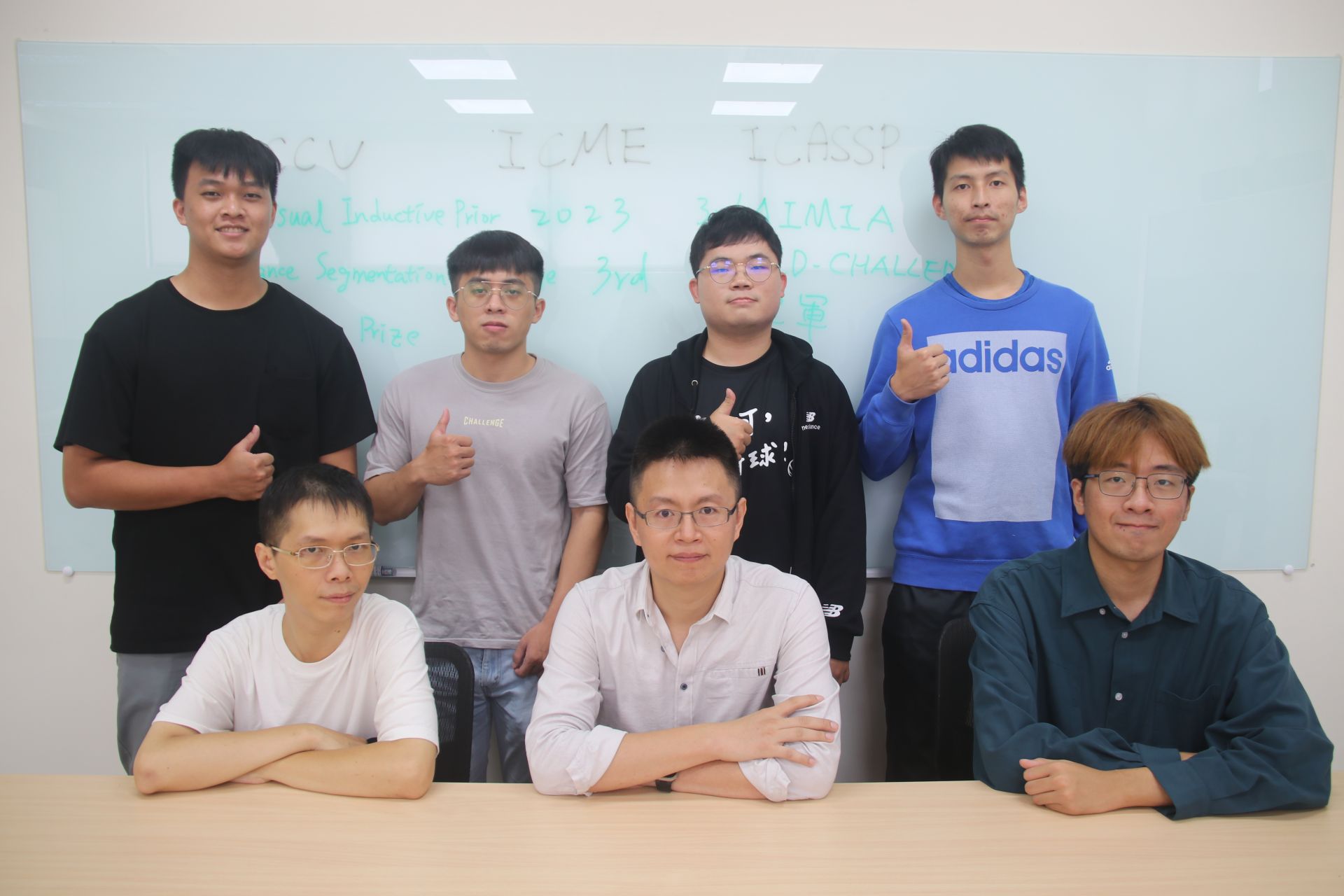
NCKU Data Science Institute ACVlab Team Dominates International Competitions with Numerous Awards Over Three Years
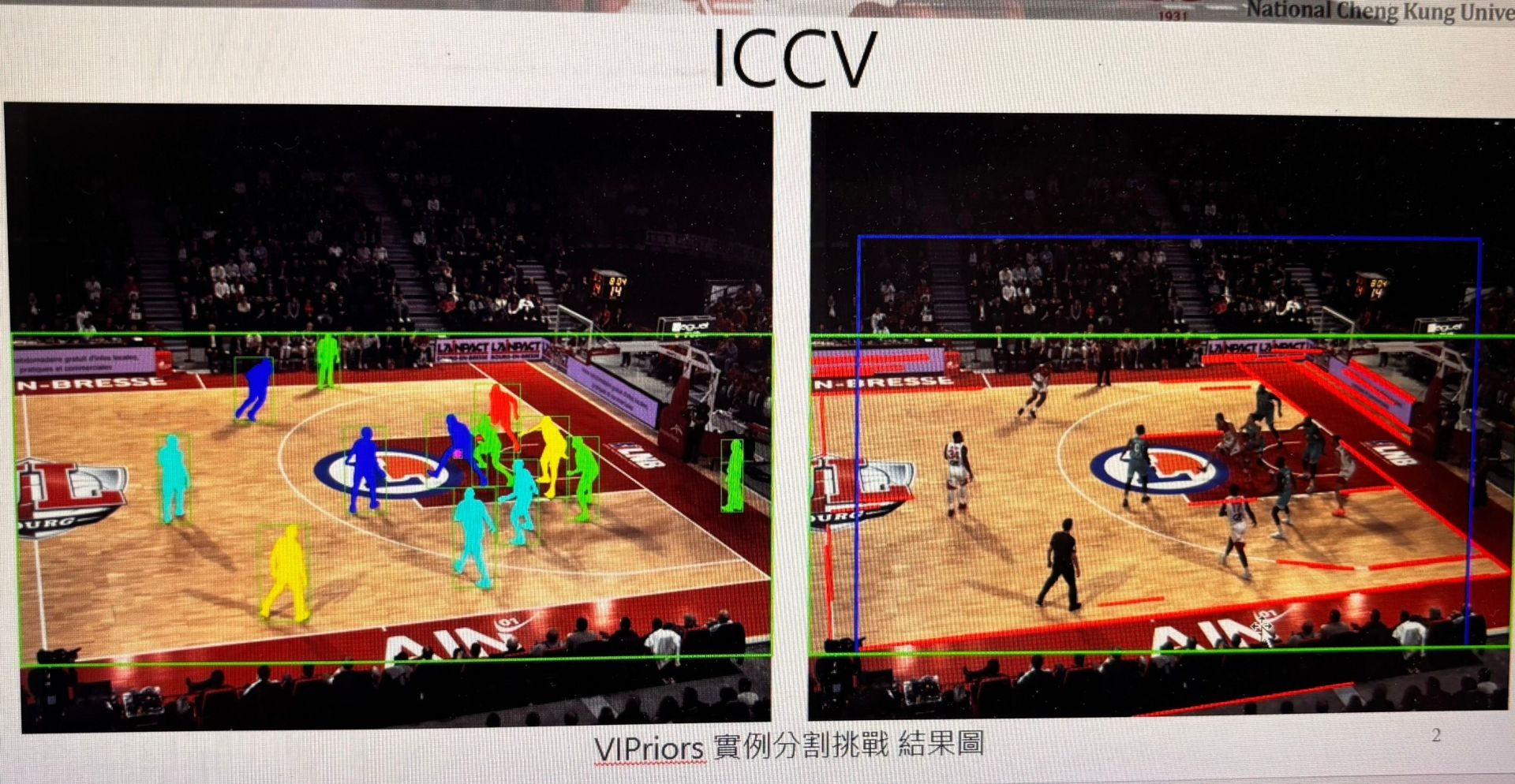
The competition task requires predicting the positions of players and the ball through a system.
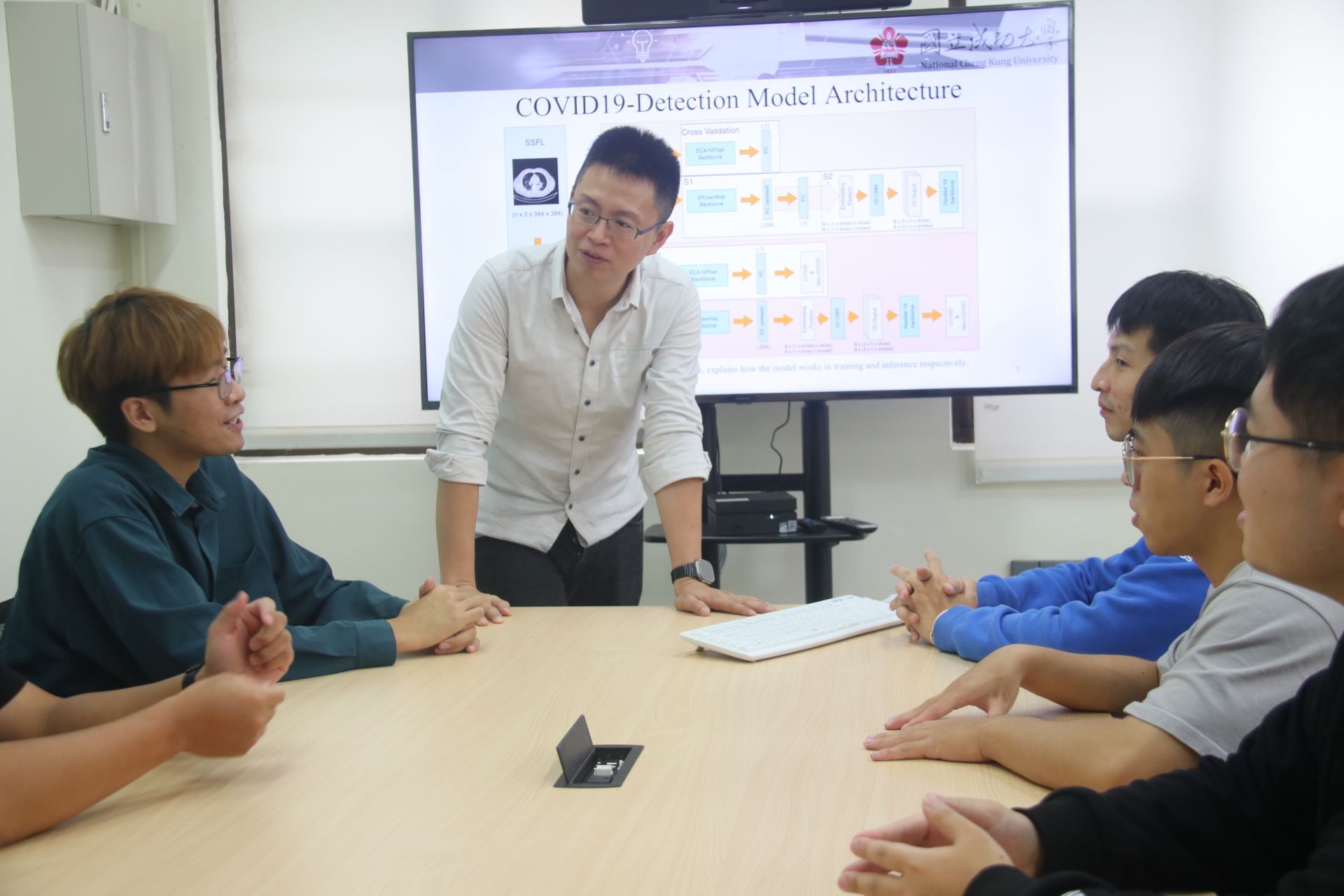
Assistant Professor Chih-Chung Hsu from the NCKU Institute of Data Science (center) established ACVlab and has led the team to participate in various international competitions over the past three years.
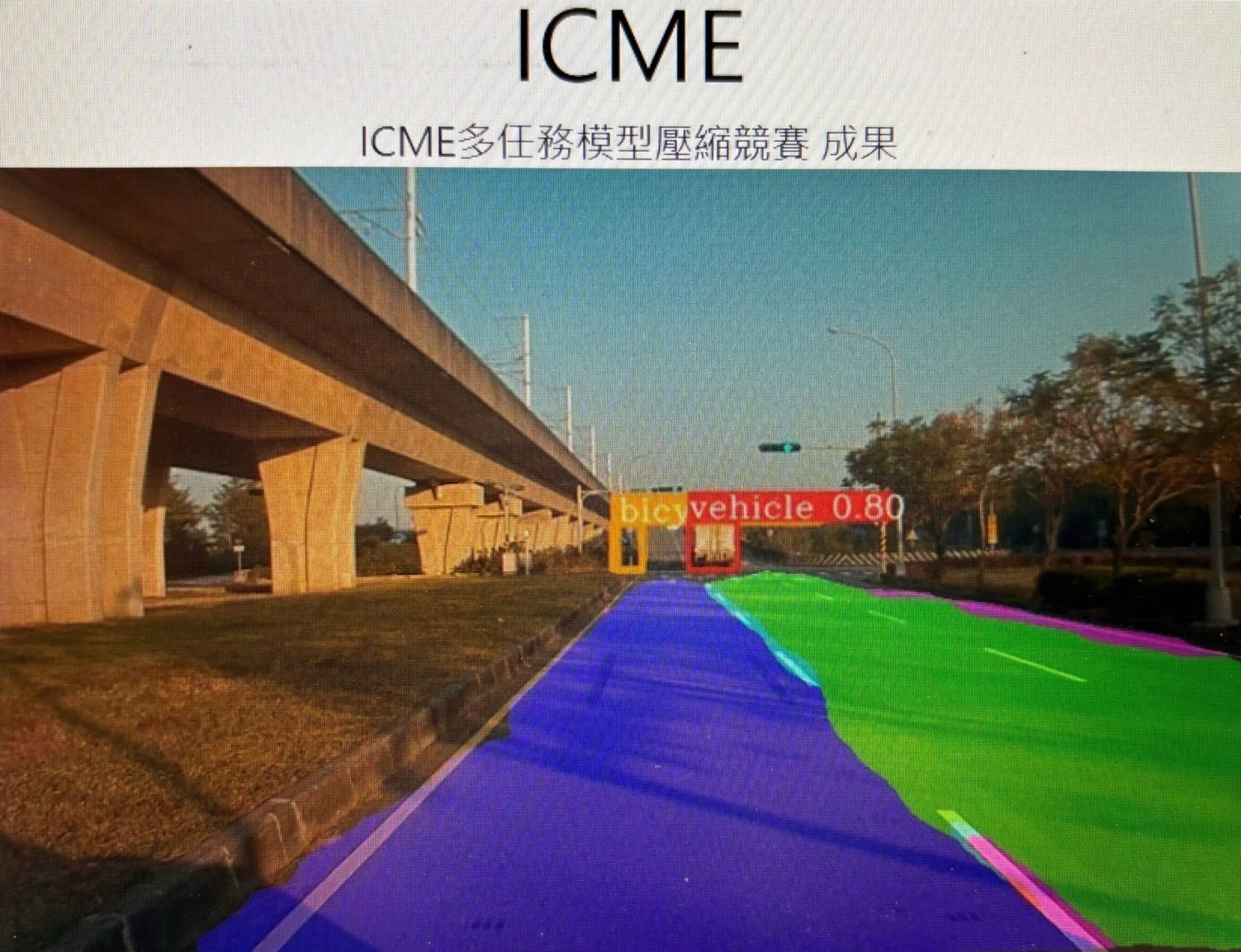
The ICME competition requires the development of a universal model that can simultaneously accomplish both semantic segmentation and object detection, two classic tasks.
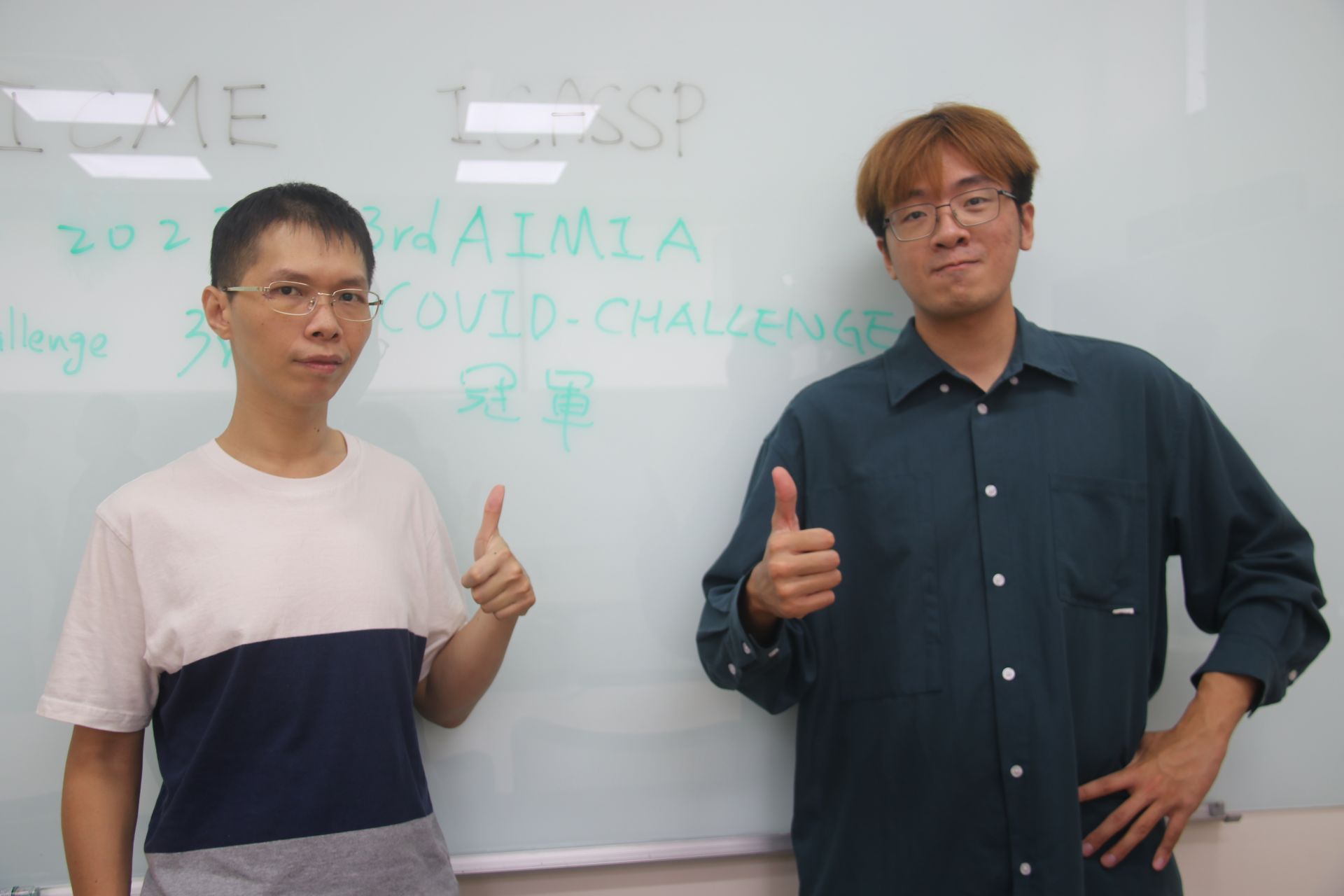
CVlab research assistant Chih-Han Tsai (left) and first-year student Chia-Ming Li from the NCKU's Institute of Data Science won the world championship at the IEEE ICASSP 3rd COV19D Competition.
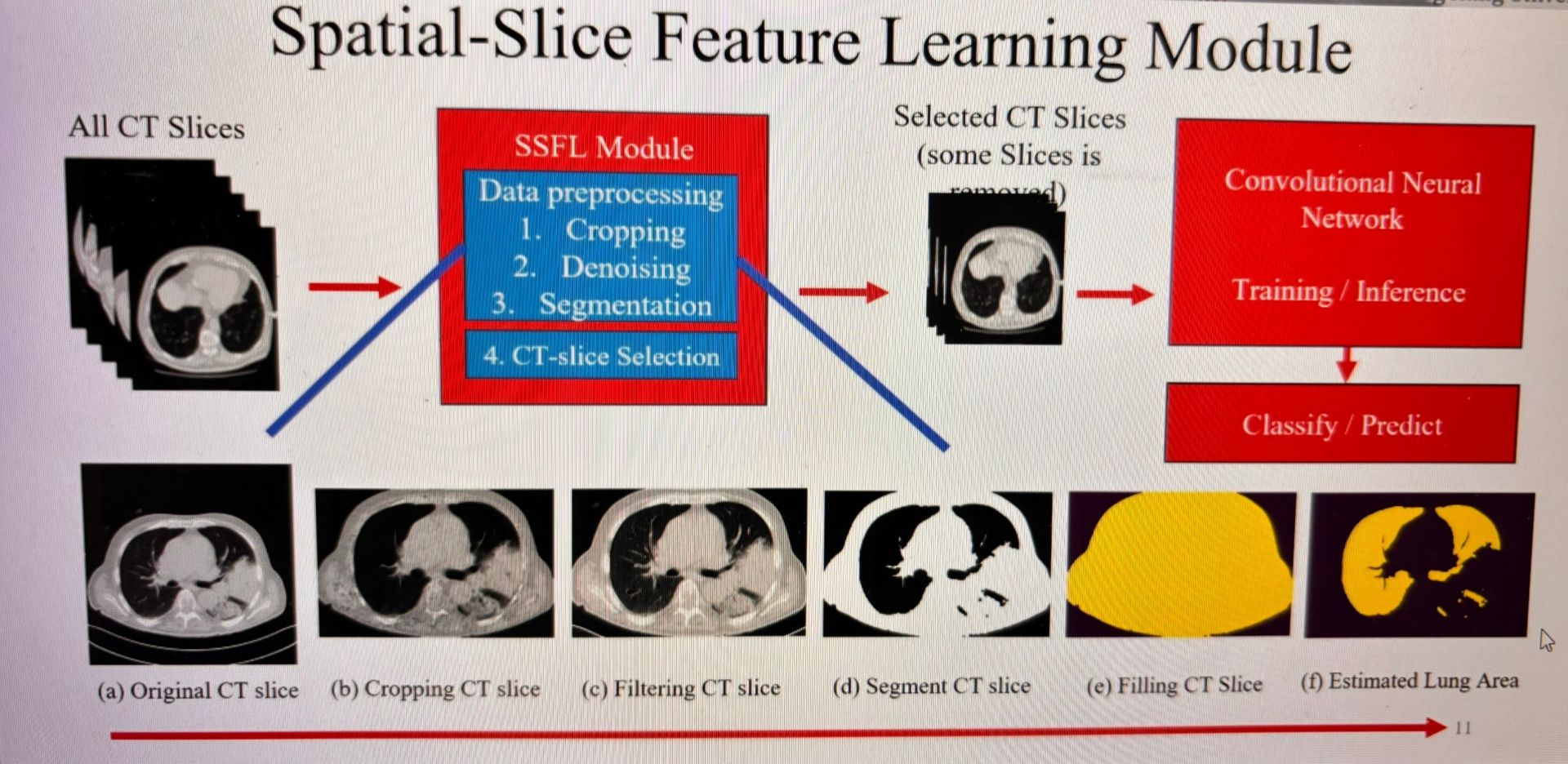
Changing the model architecture to automatically eliminate irrelevant and unimportant regions in CT images, resulting in improved detection visuals.
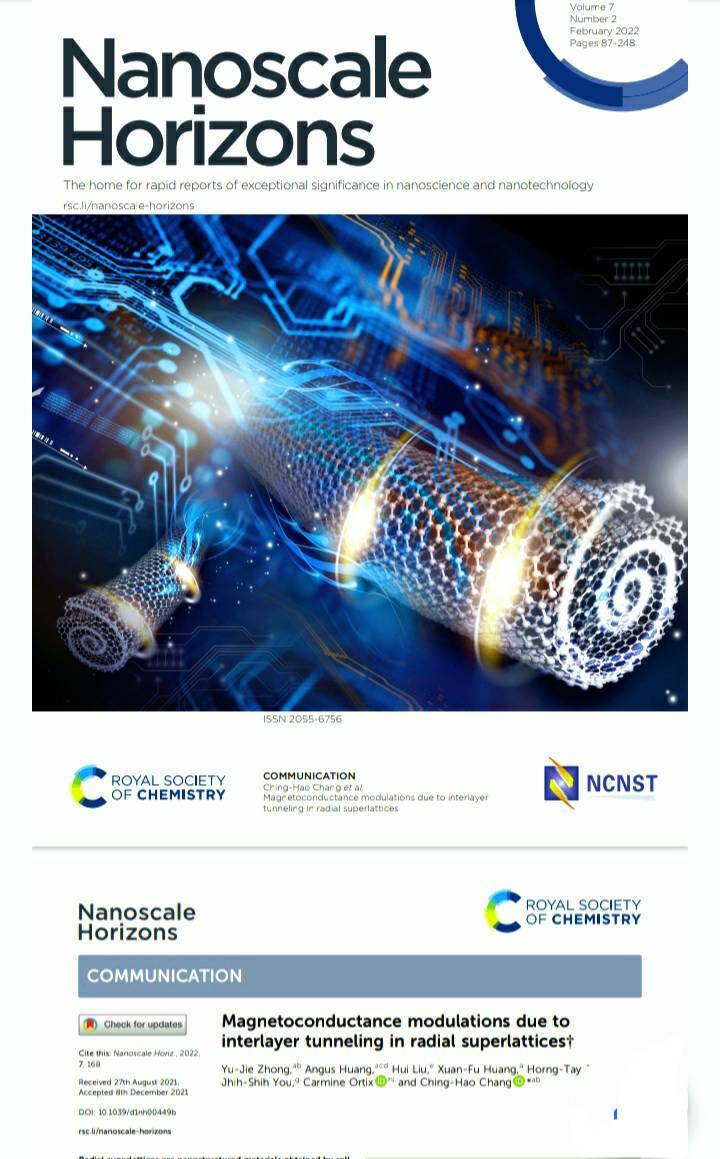
SDG9Next Generation Light and Flexible Chip Team of Ching Hao Chang Find the Key Point of Flexible Nanotechnology
View more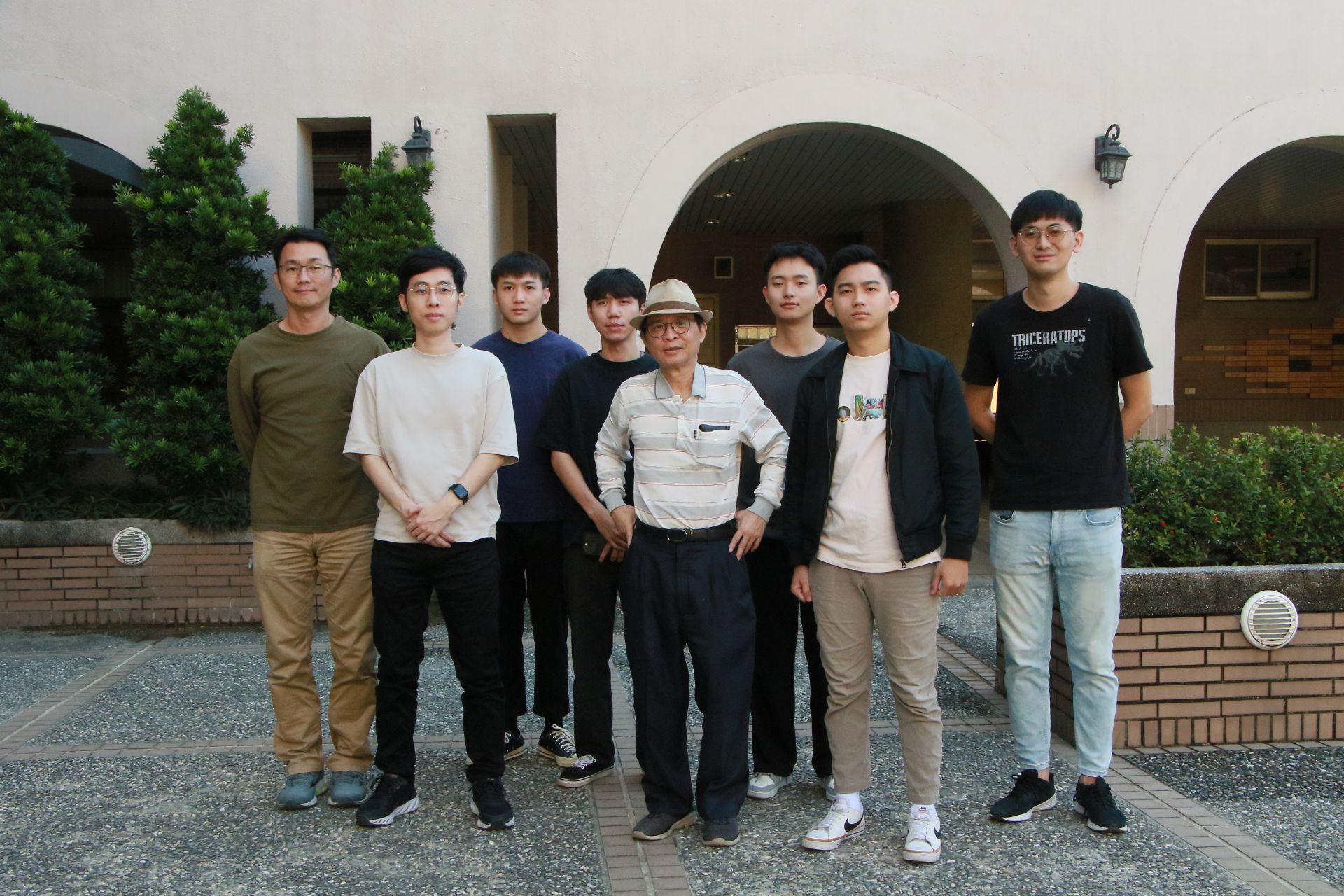
SDG9NCKU excelled in the 6th Asia-Pacific HPC-AI Student Competition 2023, winning Best AI Performance Award and Third Prize.
View more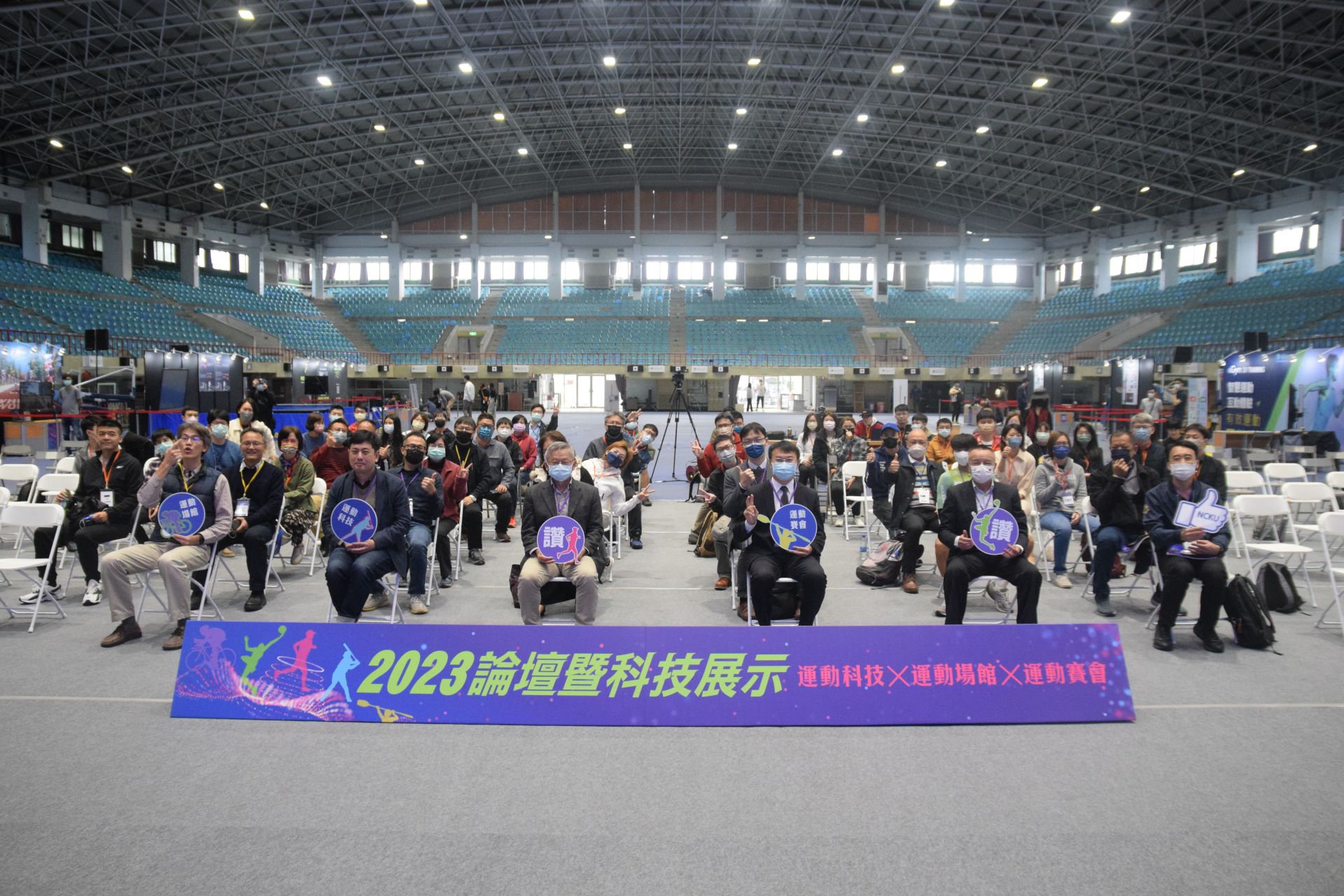