SDG9
Revolutionizing Material Design : Leveraging “Prediction + Design" AI Model in Freeze-Casting for Bioinspired Porous Materials.
Written by News Center . Image credit to Chi-Hua Yu.
While freeze-casting for bioinspired porous materials holds immense real-world application potential, the current design-to-fabrication process is still complex and fraught with uncertainties. Assistant Professor Chi-Hua Yu (Scopus) of the Department of Engineering Science at National Cheng Kung University has led a research team that successfully used deep learning models to predict the generative process and design the ice crystal structure in freeze-casting. Published in Advance Science in May, this research not only minimizes the labor and time costs involved in the design and fabrication process, but also enhances the success rate to pave the way for innovative material design. 《Advanced Science: Deep Learning Model to Predict Ice Crystal Growth》
“Freeze-casting is a burgeoning technique for fabricating bioinspired porous materials,” stated Yu. “The formation of the ice crystal structure is crucial to these porous materials' structure. Freeze-casting has been in use to create biomaterials such as biomedical bone and environmental application materials, including oil and water filtration and air filtration. However, designing the conditions for ice crystal growth has been labor and time consuming, with numerous variables which would significantly increase design complexity.”
YU: the use of deep learning and reinforcement learning
Current researches on artificial intelligence for freeze-casting have focused on predicting of the dendrite evolution to anticipate the outcome of ice crystal structure formation with conditions of different temperatures and speeds. Comparatively, Yu’s team not only employs deep learning to predict the evolution structure, but also applies reinforcement learning to impart the model with the ability to design by inputting specific target properties of the ice crystal. This new model can then devise various simulation conditions to meet the "target".
The newly developed AI model is 300,000 times faster
The "prediction + design" artificial intelligence model was independently developed by Yu and his team. ”The model drastically speeds up the ice crystal structure design process,” noted Yu. “Generally, it takes at least 5 hours for experienced designers to plan an ice crystal structure growth trend. Conversely, the artificial intelligence model can complete such tasks in just 2 to 3 minutes. As for computation costs, traditional simulation methods can take up to 2 minutes for a single simulation trial, while artificial intelligence takes only 2 seconds per inference, which is 300,000 times faster.”
Team perseverance to deal with challenges and complete the research
The research team led by Assistant Professor Chi-Hua Yu comprises Ph.D. candidate Bor-Yann Tseng, research assistant Chen-Wei Guo, and master's graduate Yu-Chen Chien. It took the team one year to complete this research, overcoming many challenges. A significant challenge was the absence of an existing AI model framework to resolve the problem. The team had to develop a new framework and designed algorithms to fulfill the research requirements. Another challenge was to effectively integrate and adjust related parameters to control the structure. Through the team's perseverance, these problems were successively resolved.
Yu: Continuous research on AI application in material science
“I will continue to lead this team to explore the application of artificial intelligence in material science and pursue advancements in this field,” stated Yu. “Currently, the porous structures of bioinspired porous materials all grow in the same direction. It would be valuable to investigate different directions of pore structure generation and consider the additive materials in the solution.”
“Advance Science” is a highly influential journal that covers research in materials science, physics, chemistry, medical and life sciences, and engineering.
While freeze-casting for bioinspired porous materials holds immense real-world application potential, the current design-to-fabrication process is still complex and fraught with uncertainties. Assistant Professor Chi-Hua Yu (Scopus) of the Department of Engineering Science at National Cheng Kung University has led a research team that successfully used deep learning models to predict the generative process and design the ice crystal structure in freeze-casting. Published in Advance Science in May, this research not only minimizes the labor and time costs involved in the design and fabrication process, but also enhances the success rate to pave the way for innovative material design. 《Advanced Science: Deep Learning Model to Predict Ice Crystal Growth》
“Freeze-casting is a burgeoning technique for fabricating bioinspired porous materials,” stated Yu. “The formation of the ice crystal structure is crucial to these porous materials' structure. Freeze-casting has been in use to create biomaterials such as biomedical bone and environmental application materials, including oil and water filtration and air filtration. However, designing the conditions for ice crystal growth has been labor and time consuming, with numerous variables which would significantly increase design complexity.”
YU: the use of deep learning and reinforcement learning
Current researches on artificial intelligence for freeze-casting have focused on predicting of the dendrite evolution to anticipate the outcome of ice crystal structure formation with conditions of different temperatures and speeds. Comparatively, Yu’s team not only employs deep learning to predict the evolution structure, but also applies reinforcement learning to impart the model with the ability to design by inputting specific target properties of the ice crystal. This new model can then devise various simulation conditions to meet the "target".
The newly developed AI model is 300,000 times faster
The "prediction + design" artificial intelligence model was independently developed by Yu and his team. ”The model drastically speeds up the ice crystal structure design process,” noted Yu. “Generally, it takes at least 5 hours for experienced designers to plan an ice crystal structure growth trend. Conversely, the artificial intelligence model can complete such tasks in just 2 to 3 minutes. As for computation costs, traditional simulation methods can take up to 2 minutes for a single simulation trial, while artificial intelligence takes only 2 seconds per inference, which is 300,000 times faster.”
Team perseverance to deal with challenges and complete the research
The research team led by Assistant Professor Chi-Hua Yu comprises Ph.D. candidate Bor-Yann Tseng, research assistant Chen-Wei Guo, and master's graduate Yu-Chen Chien. It took the team one year to complete this research, overcoming many challenges. A significant challenge was the absence of an existing AI model framework to resolve the problem. The team had to develop a new framework and designed algorithms to fulfill the research requirements. Another challenge was to effectively integrate and adjust related parameters to control the structure. Through the team's perseverance, these problems were successively resolved.
Yu: Continuous research on AI application in material science
“I will continue to lead this team to explore the application of artificial intelligence in material science and pursue advancements in this field,” stated Yu. “Currently, the porous structures of bioinspired porous materials all grow in the same direction. It would be valuable to investigate different directions of pore structure generation and consider the additive materials in the solution.”
“Advance Science” is a highly influential journal that covers research in materials science, physics, chemistry, medical and life sciences, and engineering.
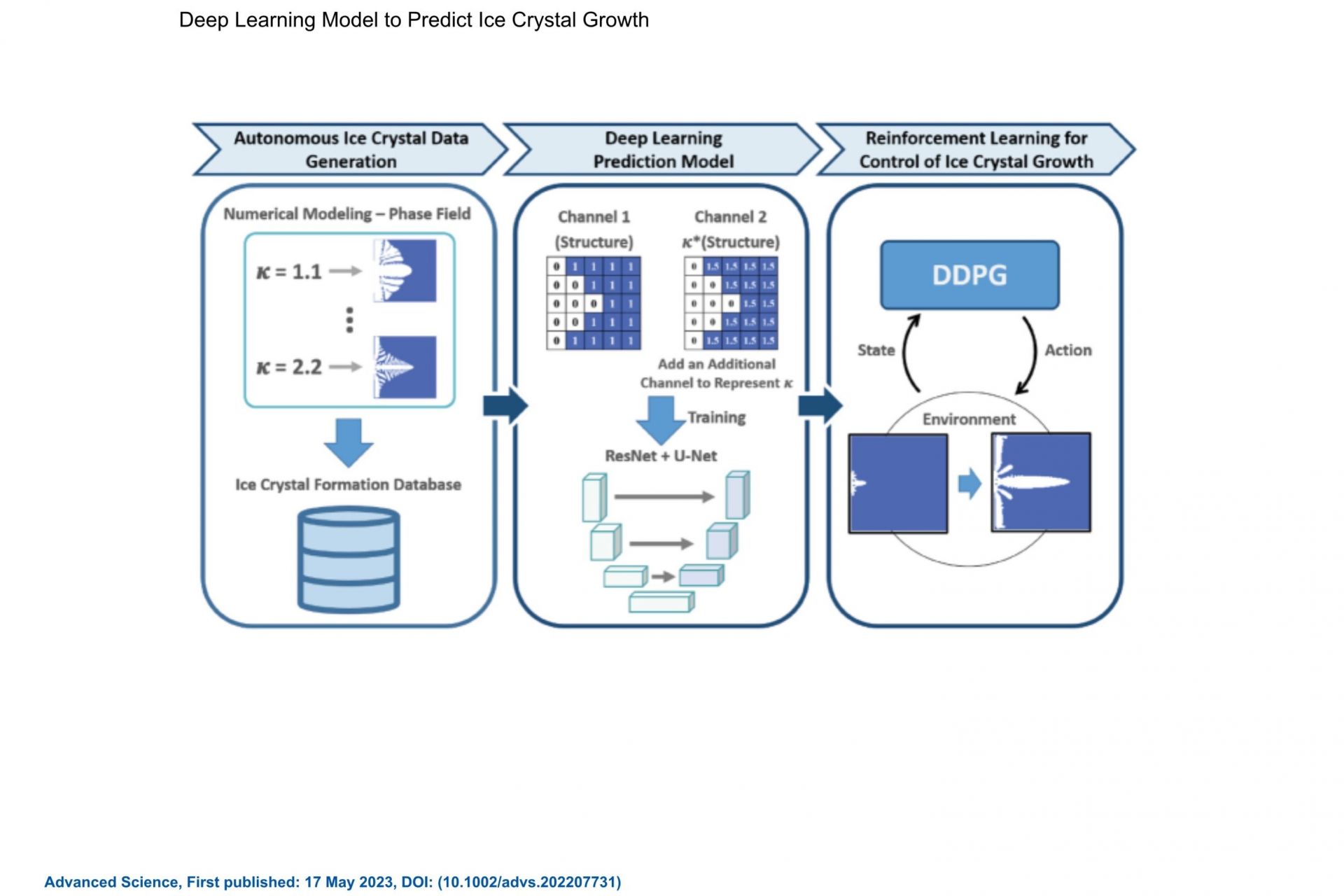
An Artificial Intelligence-based deep learning model to predict the ice crystal formation.
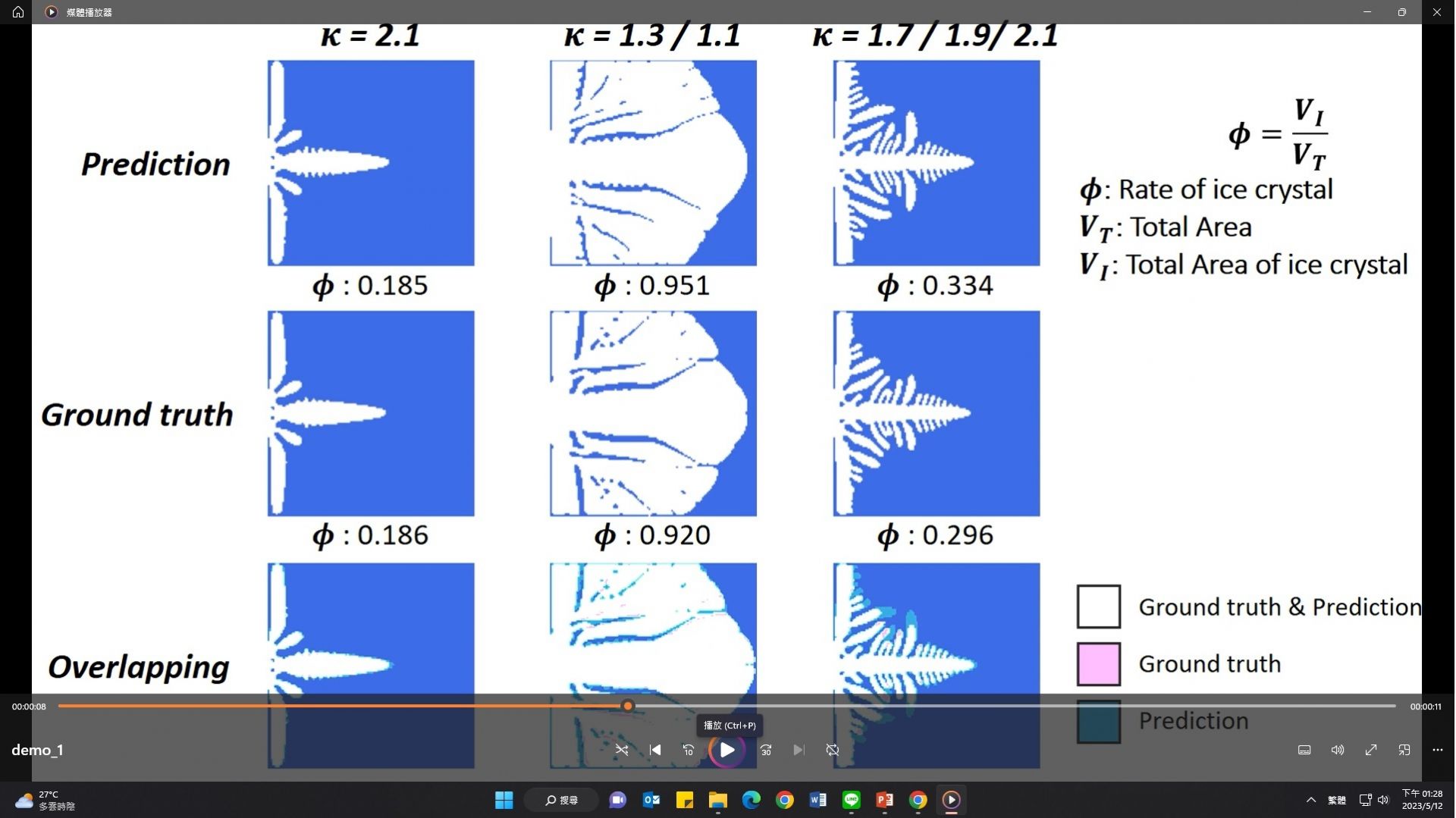
(Top to bottom) The AI-based deep learning model presents prediction of ice crystal formation, ground truth for actual growth, and the overlapping of prediction and actual growth.
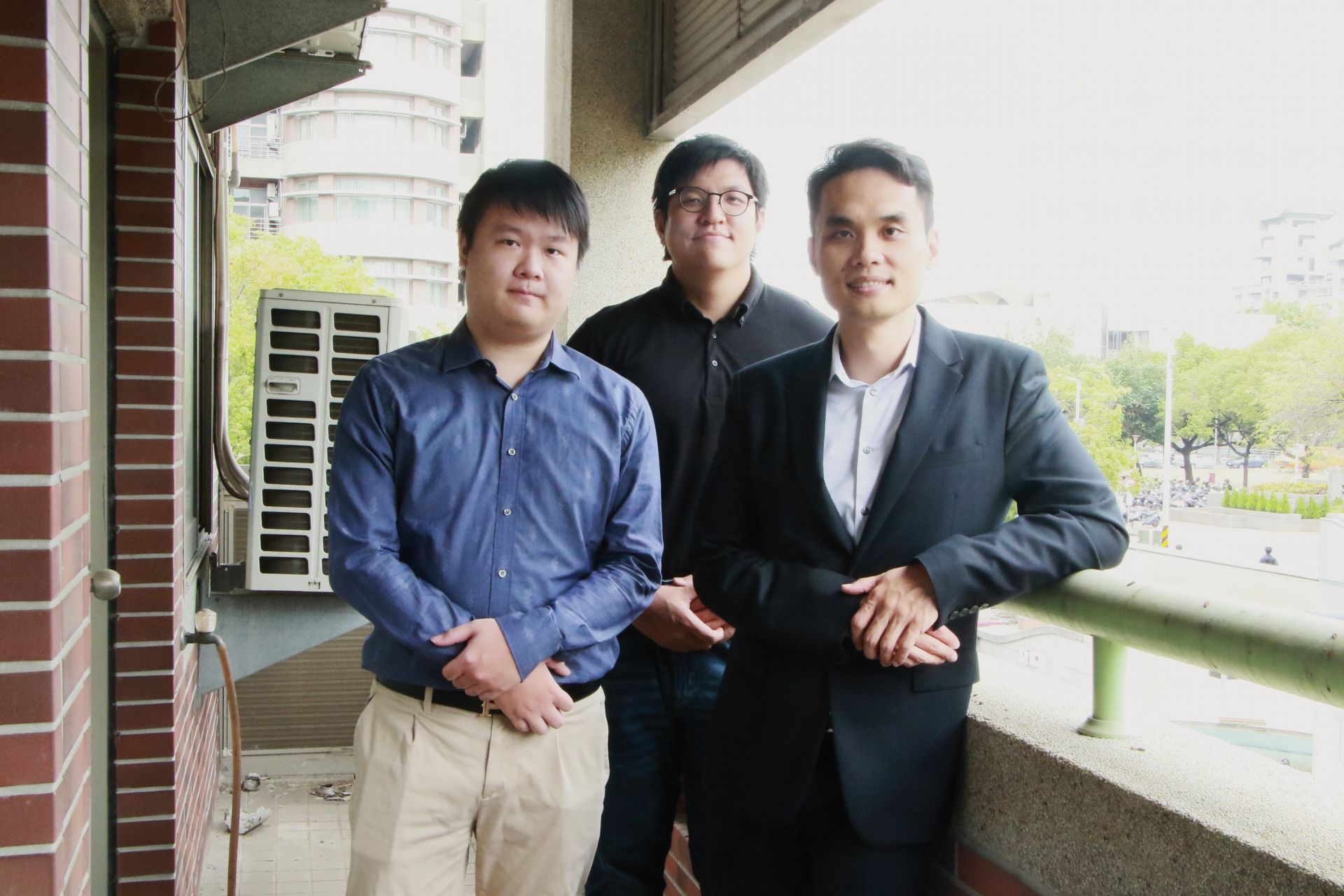
From left to right: Bor-Yann Tseng, Chen-Wei Guo and Chi-Hua Yu.
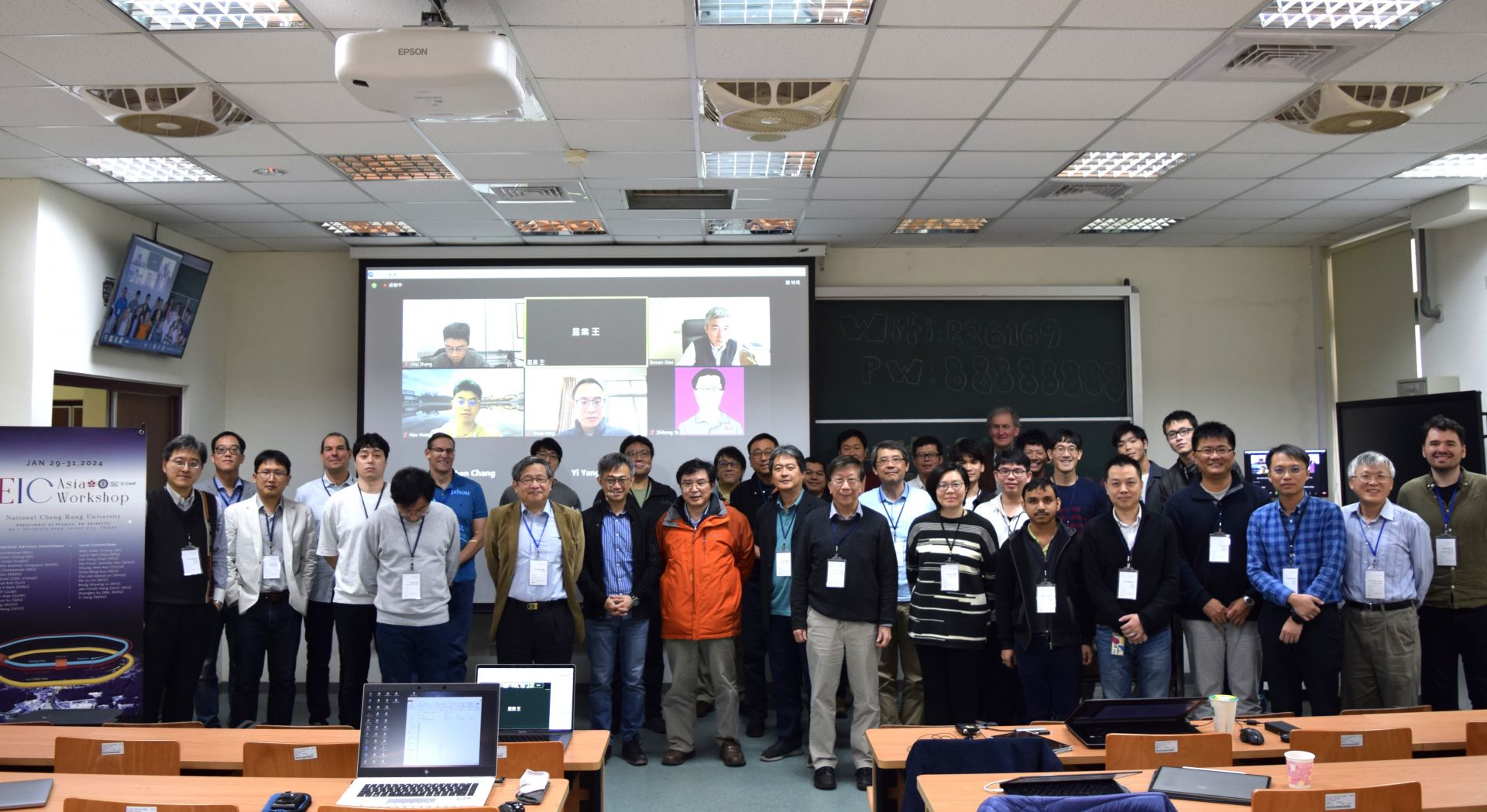
SDG9Unlocking Subatomic Mysteries: Asian Scientists Gather at NCKU to Discuss Next-Gen High-Energy Nuclear Physics
View more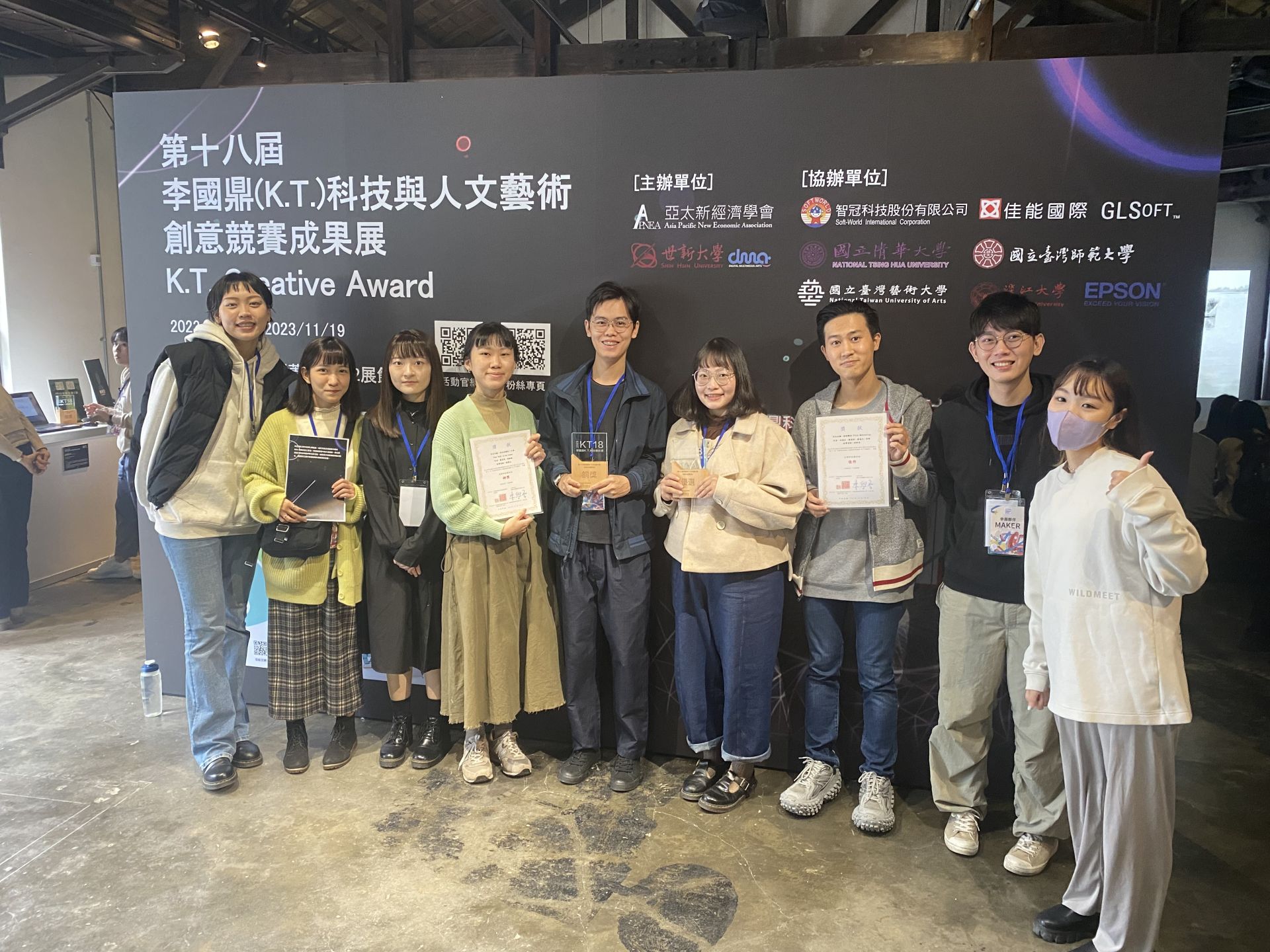
SDG9The 18th K.T. Science and Art Awards: NCKU Team Stands Out, Securing Four Honors in the Interactive Technology Art Category
View more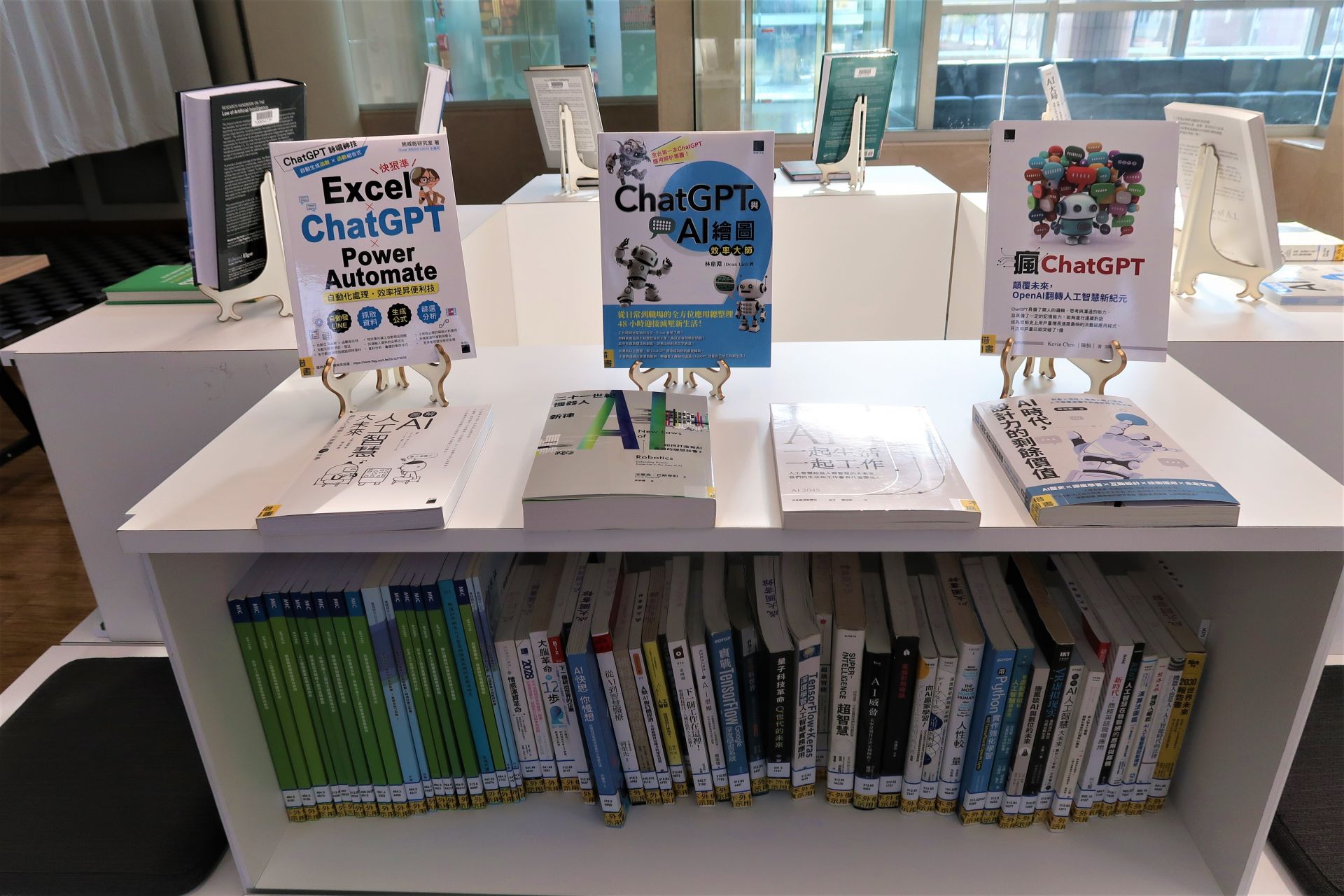