Ting-To Yu, an associate professor from the Department of Resources Engineering at NCKU, led the Earth Observing System Lab and the Earth Resources Observation Laboratory and Mineral Processing Lab, under the direction of Professor Yun-Hwei Shen and with the assistance of Professor Chi-Yen Huang. They participated in the "2010-2011 Corrugated Asbestos Cement Sheet Roof Space Distribution Estimation Baseline Survey Project" and won three national awards, including the 5th Government Service Award, the TGOS Award, and the 10th Smart City Innovation Award.
According to the survey team from the Department of Resources Engineering at NCKU, this project is the first to utilize the advantages of image recognition for machine learning and control the development of artificial intelligence to save a significant amount of manpower. They have created the "Outdoor Spatial Distribution Management System of Building Materials with Asbestos" platform to integrate the survey data into a web-based geographic information system. This has improved the administrative efficiency of data sharing and application, and allowed them to complete the difficult task of surveying all of Taiwan's asbestos cement sheet roofs in just 15 months, saving a total of 1 billion NTD.
The issue of asbestos is of great importance, and the International Programme on Chemical Safety (IPCS) of the World Health Organization (WHO) has listed it as one of the top 10 public health issues to be addressed. The Environmental Protection Agency (EPA) declared asbestos a listed toxic chemical in 1989, and the Bureau of Standards and Inspection (BSI) of the Ministry of Economic Affairs (MOEA) announced in 2006 that asbestos building materials should not contain asbestos.
The investigation team from the Department of Resources Engineering at NCKU pointed out that although asbestos building materials have been completely banned, the asbestos building materials used in the past still exist. Over time, some of the asbestos roofing panels begin to weather, and even break or crumble, which can cause the asbestos fibers to float out and scatter, posing a risk to people's health. In addition, during urban renewal, front-line workers may come into contact with asbestos building materials without proper safety isolation protection, putting their health and safety at risk.
The investigation team used machine learning to perform three types of image recognition. After using the high light spectrum to create ground truth data for approximately 500 asbestos roofs, the whole of Taiwan was divided into five regions. The 500 asbestos roofs' ground truth data were used as training data for the first tier of aerial and satellite photos. After verification, the first-tier roofs were used as the second-tier image training samples, and the AI machine learning characteristics were used to gradually complete the interpretation of the entire Taiwan asbestos tile roofs by analogy. They also used multi-temporal Google Street View data and UAV data for follow-up verification.
Through the results of image interpretation, they have built the "Outdoor Spatial Distribution Management System for Building Materials Containing Asbestos" platform to integrate the survey data into a web-based version of the Geographic Information System. This platform can be used online for cross-unit use of related editorial information, gradually expanding from the original basic attributes to subsequent reporting, in-situ clearance processing, and related temporal data updates. This allows central and local authorities to browse overall, regional, or individual data and related statistical information through the system platform, achieving central and local data sharing and application management purposes, assisting administrative needs, and improving the results of the three major directions.
The project began in 2010 with the launch of a telemetry interpretation service for the census of asbestos roofs. This service linked a vast amount of government and non-government data, integrated multi-scale telemetry data, and employed AI and machine learning methods to automatically interpret the spatial distribution of asbestos roofs. The interpretation was then verified through qualitative and quantitative inspection by qualified asbestos inspection laboratories, following automatic image interpretation and manual checking and editing. With innovative digital technology, the baseline survey of all Taiwan's asbestos tile roofs was completed. The results of the phased sampling tests showed an overall accuracy rate of over 90%. This solution addresses the long-standing problem of not being able to accurately estimate the number of asbestos roofs nationwide. The project effectively integrates cross-agency resources to construct high-quality and user-friendly information on the spatial distribution of asbestos roofs throughout Taiwan. This information provides the central ministry and local environmental protection bureaus with timely information for demolition management, inspection, evaluation, subsidy for replacement, and removal and disposal of asbestos-containing waste. The project serves as the basis for government services, meeting the expectations of society.
According to the survey team from the Department of Resources Engineering at NCKU, this project is the first to utilize the advantages of image recognition for machine learning and control the development of artificial intelligence to save a significant amount of manpower. They have created the "Outdoor Spatial Distribution Management System of Building Materials with Asbestos" platform to integrate the survey data into a web-based geographic information system. This has improved the administrative efficiency of data sharing and application, and allowed them to complete the difficult task of surveying all of Taiwan's asbestos cement sheet roofs in just 15 months, saving a total of 1 billion NTD.
The issue of asbestos is of great importance, and the International Programme on Chemical Safety (IPCS) of the World Health Organization (WHO) has listed it as one of the top 10 public health issues to be addressed. The Environmental Protection Agency (EPA) declared asbestos a listed toxic chemical in 1989, and the Bureau of Standards and Inspection (BSI) of the Ministry of Economic Affairs (MOEA) announced in 2006 that asbestos building materials should not contain asbestos.
The investigation team from the Department of Resources Engineering at NCKU pointed out that although asbestos building materials have been completely banned, the asbestos building materials used in the past still exist. Over time, some of the asbestos roofing panels begin to weather, and even break or crumble, which can cause the asbestos fibers to float out and scatter, posing a risk to people's health. In addition, during urban renewal, front-line workers may come into contact with asbestos building materials without proper safety isolation protection, putting their health and safety at risk.
The investigation team used machine learning to perform three types of image recognition. After using the high light spectrum to create ground truth data for approximately 500 asbestos roofs, the whole of Taiwan was divided into five regions. The 500 asbestos roofs' ground truth data were used as training data for the first tier of aerial and satellite photos. After verification, the first-tier roofs were used as the second-tier image training samples, and the AI machine learning characteristics were used to gradually complete the interpretation of the entire Taiwan asbestos tile roofs by analogy. They also used multi-temporal Google Street View data and UAV data for follow-up verification.
Through the results of image interpretation, they have built the "Outdoor Spatial Distribution Management System for Building Materials Containing Asbestos" platform to integrate the survey data into a web-based version of the Geographic Information System. This platform can be used online for cross-unit use of related editorial information, gradually expanding from the original basic attributes to subsequent reporting, in-situ clearance processing, and related temporal data updates. This allows central and local authorities to browse overall, regional, or individual data and related statistical information through the system platform, achieving central and local data sharing and application management purposes, assisting administrative needs, and improving the results of the three major directions.
The project began in 2010 with the launch of a telemetry interpretation service for the census of asbestos roofs. This service linked a vast amount of government and non-government data, integrated multi-scale telemetry data, and employed AI and machine learning methods to automatically interpret the spatial distribution of asbestos roofs. The interpretation was then verified through qualitative and quantitative inspection by qualified asbestos inspection laboratories, following automatic image interpretation and manual checking and editing. With innovative digital technology, the baseline survey of all Taiwan's asbestos tile roofs was completed. The results of the phased sampling tests showed an overall accuracy rate of over 90%. This solution addresses the long-standing problem of not being able to accurately estimate the number of asbestos roofs nationwide. The project effectively integrates cross-agency resources to construct high-quality and user-friendly information on the spatial distribution of asbestos roofs throughout Taiwan. This information provides the central ministry and local environmental protection bureaus with timely information for demolition management, inspection, evaluation, subsidy for replacement, and removal and disposal of asbestos-containing waste. The project serves as the basis for government services, meeting the expectations of society.
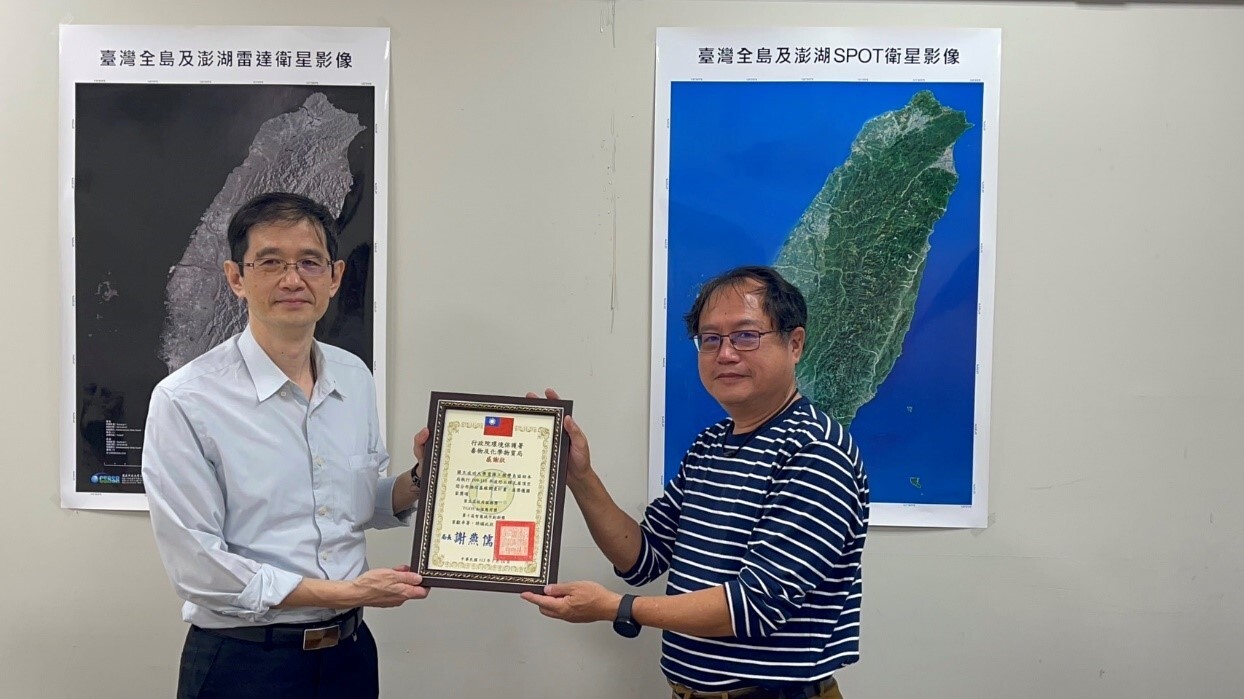
Yi-Kuen Liu, a Division Chief from Toxic and Chemical Substances Bureau presented a certificate of appreciation to the team of NCKU, and was received by Prof. Ting-To Yu
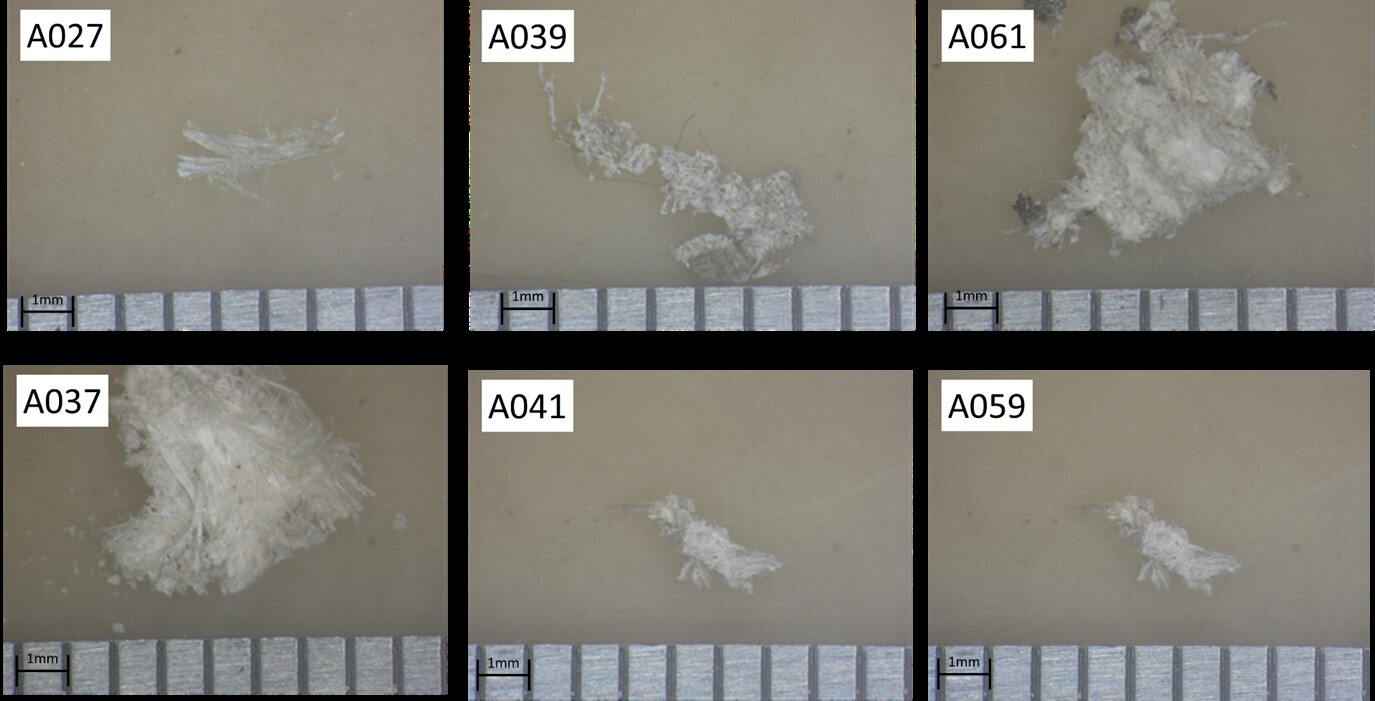
Qualitative sample of asbestos cement sheet: Asbestos fiber extracted after acid washing
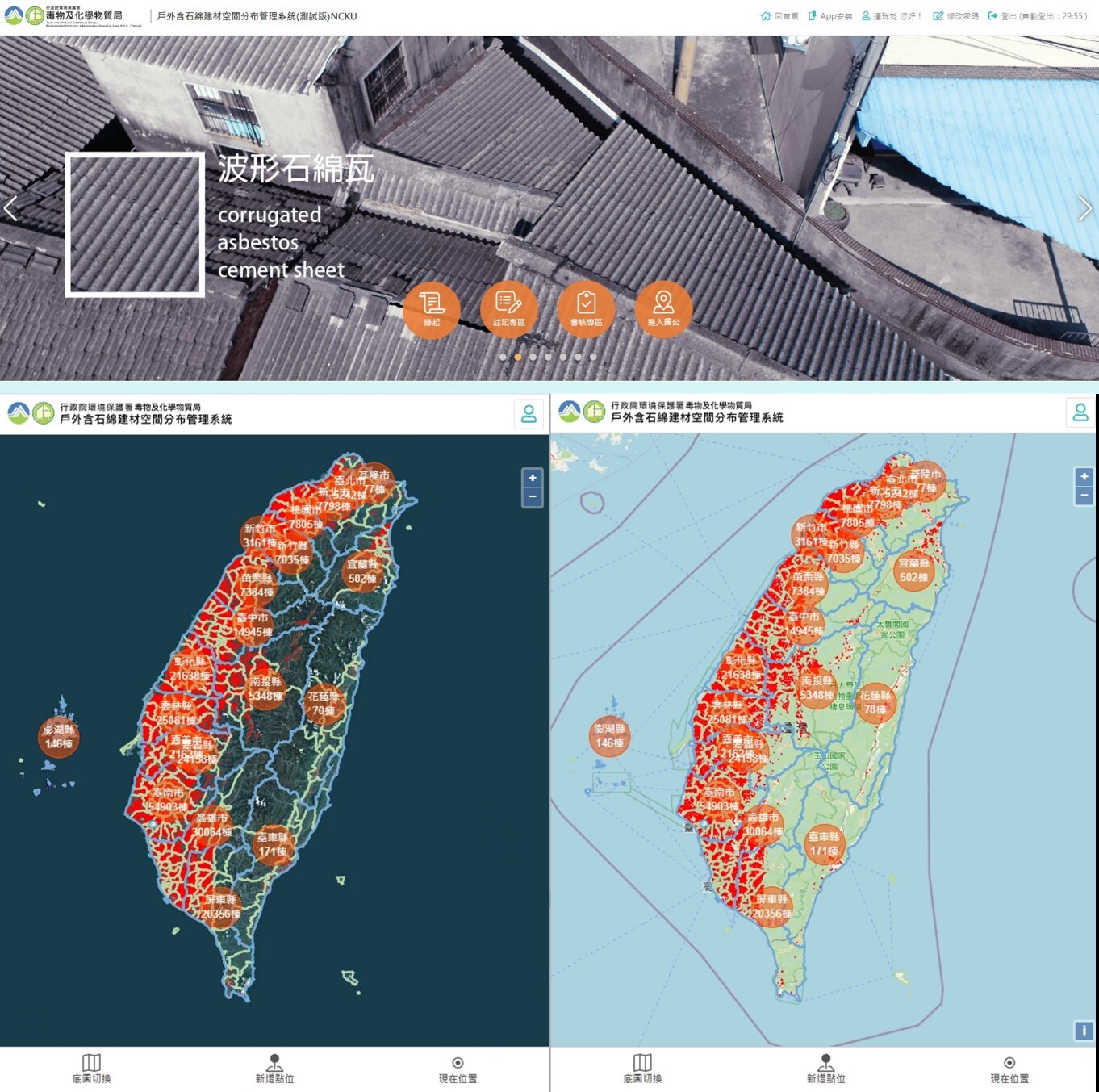
The "Outdoor Spatial Distribution Management System for Building Materials Containing Asbestos" Platform