SDG11
AI Helps Protect the Environment: NCKU Resource Engineering Asbestos Roofing Remote Sensing Team Wins 3 National Awards.
National Cheng Kung University's (NCKU) Department of Resources Engineering Associate Professor Ting-To Yu led the Earth Resource Observation Laboratory, along with Professor Yun-Hwei Shen's Ore Dressing Laboratory under his guidance and assistance from Professor Ji-Yan Huang, in participating in the "Baseline Survey of Asbestos Roof Spatial Distribution for the Years 2020-2021". The team has received three national awards: the Fifth Government Service Award, the TGOS Value-added Application Award, and the Tenth Smart City Innovation Award.
The investigative team from NCKU's Department of Resources Engineering stated that this project was a pioneer in utilizing artificial intelligence (AI) development. By leveraging the advantages of machine learning in three major image recognition techniques, it significantly reduced the need for manual labor in surveys. They also developed the "Outdoor Space Distribution Management System for Asbestos-containing Building Materials," integrating survey data into a web-based Geographic Information System (GIS) platform to enhance administrative management efficiency, leading to a significant cost savings of approximately 1 billion NTD over 15 months for the nationwide asbestos roof survey.
The issue of asbestos is of paramount importance, with the World Health Organization's International Programme on Chemical Safety listing asbestos among the top ten public health concerns. The Environmental Protection Administration classified asbestos as a toxic chemical substance in 1989, and the Bureau of Standards, Metrology & Inspection announced in 2006 that asbestos-containing building materials were prohibited.
While asbestos-containing building materials have been banned, remnants from past usage still persist. Over time, these materials, especially asbestos wave tiles used on roofs, deteriorate and may even become fractured or fragmented, releasing asbestos fibers into the air. Asbestos fibers are extremely small, posing health risks to people living in proximity. Additionally, during urban renewal projects, workers involved in demolishing asbestos-containing old buildings or renovating structures may be exposed to asbestos materials without adequate safety precautions, jeopardizing their health and safety.
The investigative team from NCKU's Department of Resources Engineering utilized machine learning for image recognition in three phases. They initially established approximately 500 locations of asbestos wave tile roofs using hyperspectral imaging as the first-phase training data for aerial and satellite imagery. Subsequently, they used the results of the first phase for further interpretation, followed by validation. The interpreted asbestos wave tile roofs from the first phase were then used as training samples for the second phase of image interpretation. This process was repeated, gradually completing the interpretation of asbestos wave tile roofs across Taiwan.
Furthermore, to overcome limitations posed by external environmental conditions during surveys, the team utilized multiple-time series of Google Street View data and UAV imagery for subsequent validation and confirmation, realizing the transition from visual inspection to remote sensing, thus enabling a perspective of Taiwan from space. In addition to basic survey costs, further expenses may amount to hundreds of millions of NTD for data compilation, organization, and verification.
Based on the results of image interpretation, the team established the "Outdoor Space Distribution Management System for Asbestos-containing Building Materials" platform, integrating survey data into a web-based GIS platform. The platform allows cross-agency access to relevant editing information online, expanding from basic attributes to subsequent declaration, on-site removal processing, and updates on relevant time-series data. Central and local government agencies can access the overall, zonal, or individual data and related statistical information through the system platform, achieving the goal of data sharing and application management between central and local authorities, thus assisting in administrative management needs and enhancing three major aspects of outcomes.
This project commenced aerial remote sensing for asbestos roof surveys in 2020, linking massive data from government and private sectors. By integrating multiscale remote sensing data and utilizing AI and machine learning methods for automatic interpretation of the spatial distribution of asbestos roof tiles, combined with qualitative and quantitative testing verification in qualified asbestos testing laboratories, image automatic interpretation followed by manual verification and digitization achieved a baseline survey of asbestos roof tiles nationwide. The overall correct interpretation rate exceeded 90%, addressing the long-standing challenge of statistically determining the nationwide quantity of asbestos roof tiles. It effectively integrated and interfaced cross-agency resources, constructing high-quality, user-friendly spatial distribution location data of asbestos roof tiles nationwide, and timely providing central ministries and local environmental protection bureaus with policy-making foundations for promoting demolition management, verification and inspection, evaluation subsidies for replacement, and clearance processing of asbestos-containing waste, meeting societal expectations.
The investigative team from NCKU's Department of Resources Engineering stated that this project was a pioneer in utilizing artificial intelligence (AI) development. By leveraging the advantages of machine learning in three major image recognition techniques, it significantly reduced the need for manual labor in surveys. They also developed the "Outdoor Space Distribution Management System for Asbestos-containing Building Materials," integrating survey data into a web-based Geographic Information System (GIS) platform to enhance administrative management efficiency, leading to a significant cost savings of approximately 1 billion NTD over 15 months for the nationwide asbestos roof survey.
The issue of asbestos is of paramount importance, with the World Health Organization's International Programme on Chemical Safety listing asbestos among the top ten public health concerns. The Environmental Protection Administration classified asbestos as a toxic chemical substance in 1989, and the Bureau of Standards, Metrology & Inspection announced in 2006 that asbestos-containing building materials were prohibited.
While asbestos-containing building materials have been banned, remnants from past usage still persist. Over time, these materials, especially asbestos wave tiles used on roofs, deteriorate and may even become fractured or fragmented, releasing asbestos fibers into the air. Asbestos fibers are extremely small, posing health risks to people living in proximity. Additionally, during urban renewal projects, workers involved in demolishing asbestos-containing old buildings or renovating structures may be exposed to asbestos materials without adequate safety precautions, jeopardizing their health and safety.
The investigative team from NCKU's Department of Resources Engineering utilized machine learning for image recognition in three phases. They initially established approximately 500 locations of asbestos wave tile roofs using hyperspectral imaging as the first-phase training data for aerial and satellite imagery. Subsequently, they used the results of the first phase for further interpretation, followed by validation. The interpreted asbestos wave tile roofs from the first phase were then used as training samples for the second phase of image interpretation. This process was repeated, gradually completing the interpretation of asbestos wave tile roofs across Taiwan.
Furthermore, to overcome limitations posed by external environmental conditions during surveys, the team utilized multiple-time series of Google Street View data and UAV imagery for subsequent validation and confirmation, realizing the transition from visual inspection to remote sensing, thus enabling a perspective of Taiwan from space. In addition to basic survey costs, further expenses may amount to hundreds of millions of NTD for data compilation, organization, and verification.
Based on the results of image interpretation, the team established the "Outdoor Space Distribution Management System for Asbestos-containing Building Materials" platform, integrating survey data into a web-based GIS platform. The platform allows cross-agency access to relevant editing information online, expanding from basic attributes to subsequent declaration, on-site removal processing, and updates on relevant time-series data. Central and local government agencies can access the overall, zonal, or individual data and related statistical information through the system platform, achieving the goal of data sharing and application management between central and local authorities, thus assisting in administrative management needs and enhancing three major aspects of outcomes.
This project commenced aerial remote sensing for asbestos roof surveys in 2020, linking massive data from government and private sectors. By integrating multiscale remote sensing data and utilizing AI and machine learning methods for automatic interpretation of the spatial distribution of asbestos roof tiles, combined with qualitative and quantitative testing verification in qualified asbestos testing laboratories, image automatic interpretation followed by manual verification and digitization achieved a baseline survey of asbestos roof tiles nationwide. The overall correct interpretation rate exceeded 90%, addressing the long-standing challenge of statistically determining the nationwide quantity of asbestos roof tiles. It effectively integrated and interfaced cross-agency resources, constructing high-quality, user-friendly spatial distribution location data of asbestos roof tiles nationwide, and timely providing central ministries and local environmental protection bureaus with policy-making foundations for promoting demolition management, verification and inspection, evaluation subsidies for replacement, and clearance processing of asbestos-containing waste, meeting societal expectations.
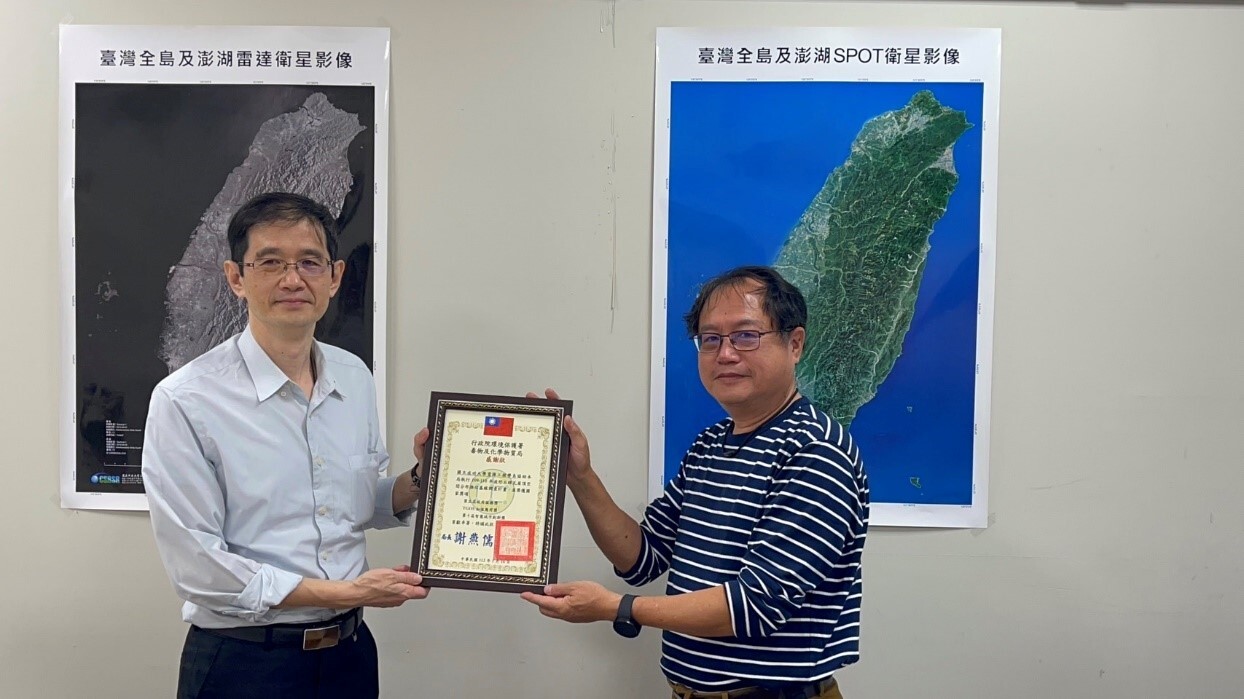
Chemical Bureau Chief Yi-kun Liu (left) of the Environmental Protection Administration presents a certificate of appreciation to Associate Professor Ting-To Yu (right) from the Department of Resources Engineering, representing the team.
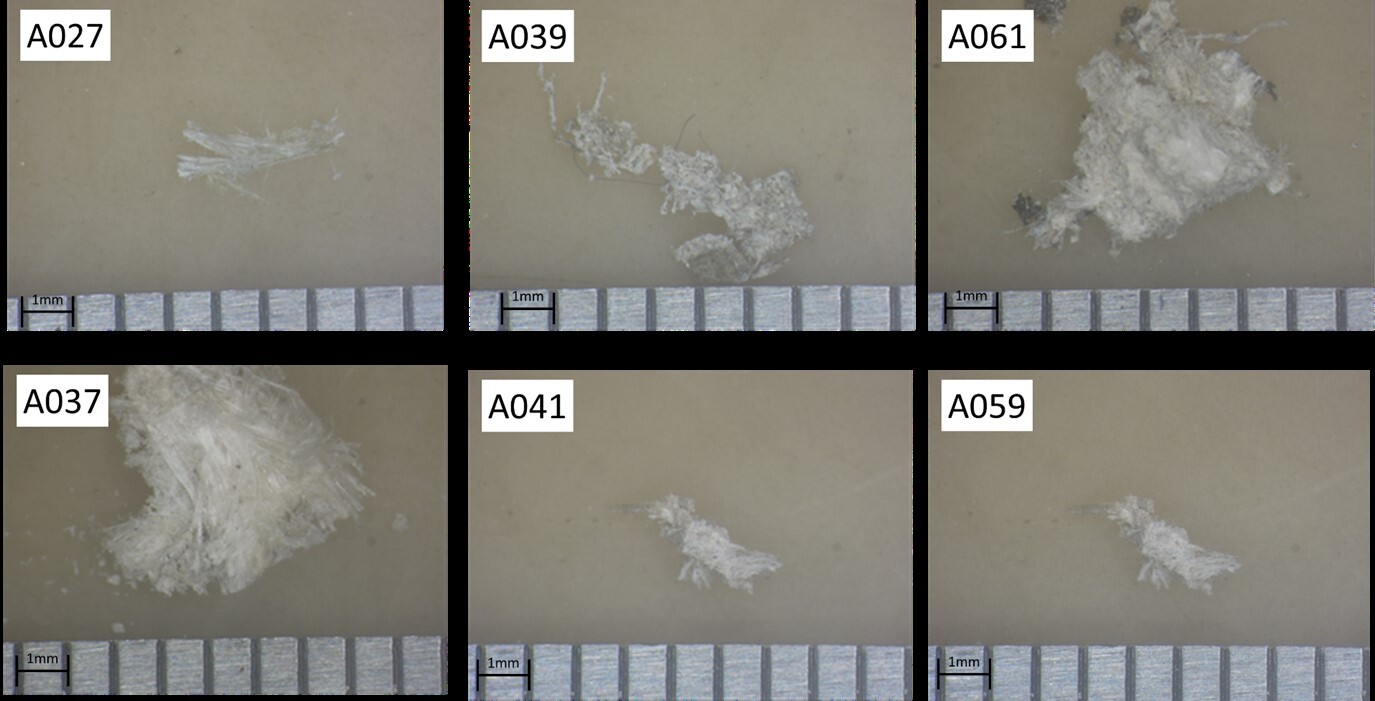
Asbestos tile qualitative sample: Asbestos fibers extracted after acid washing.
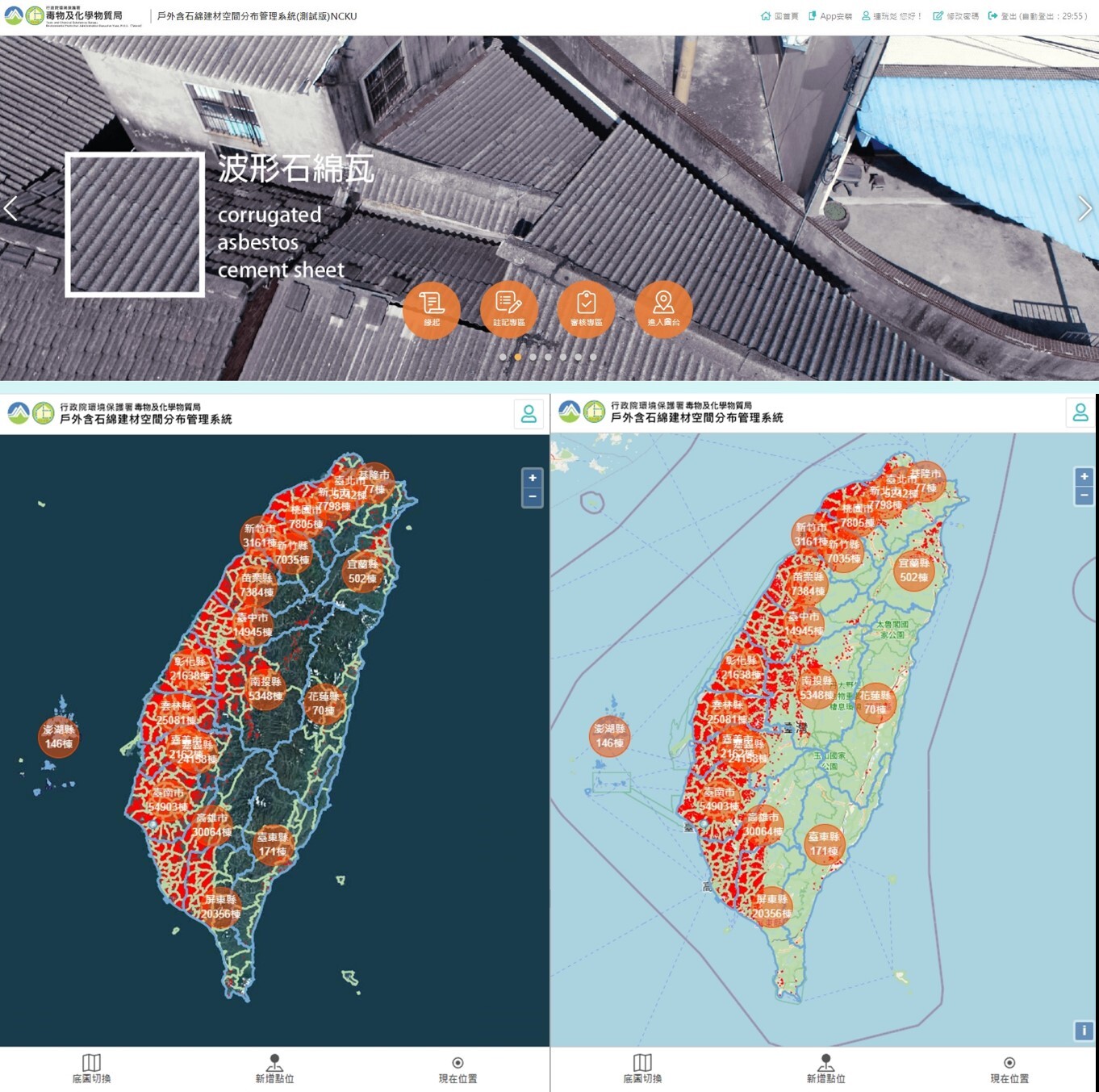
"Outdoor Asbestos-Containing Building Materials Spatial Distribution Management System" Platform Display Interface.
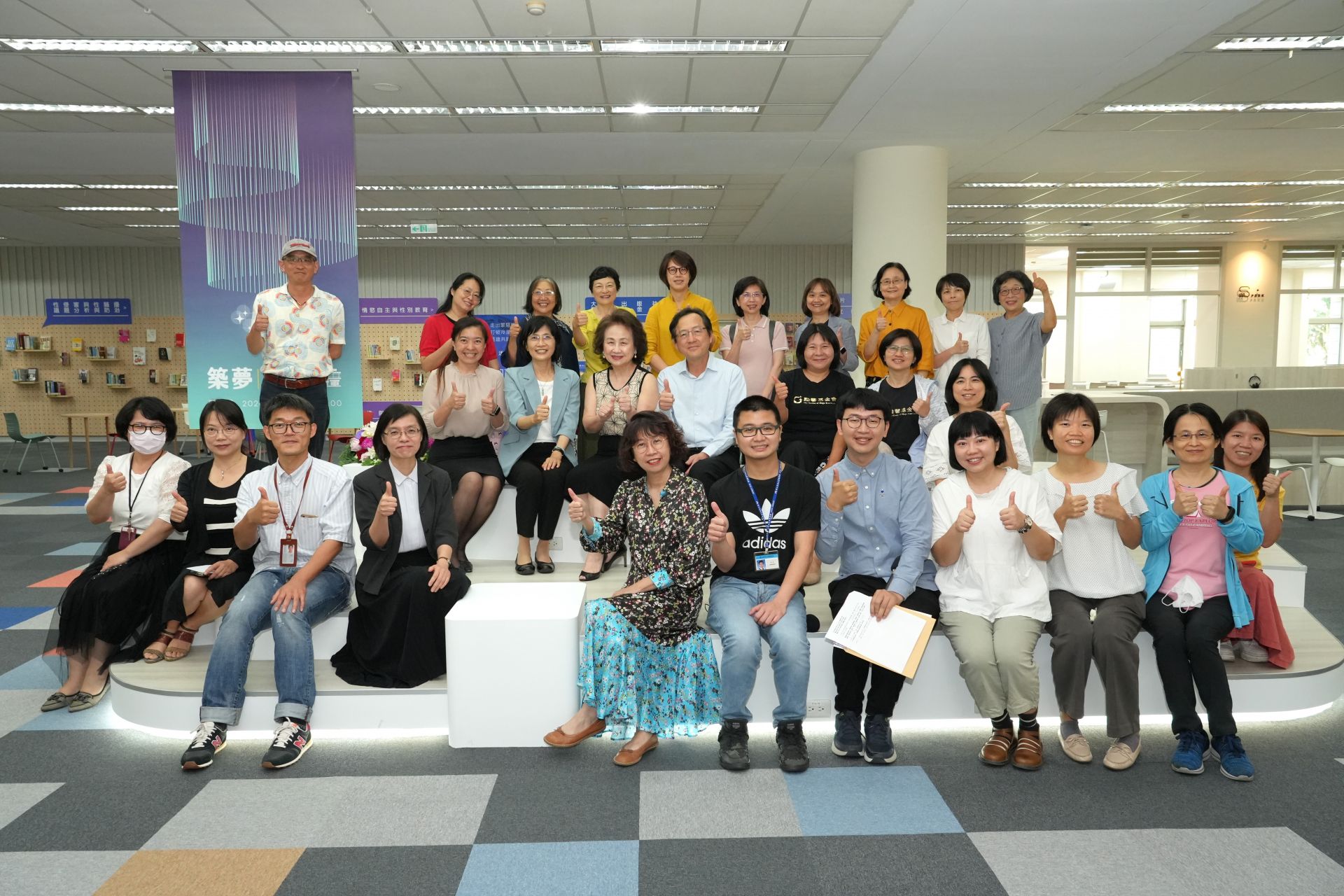
SDG11Dream into Reality, Building Dreams Solidly: Completion and Inauguration of Phase Two Space Transformation at NCKU Library.
View more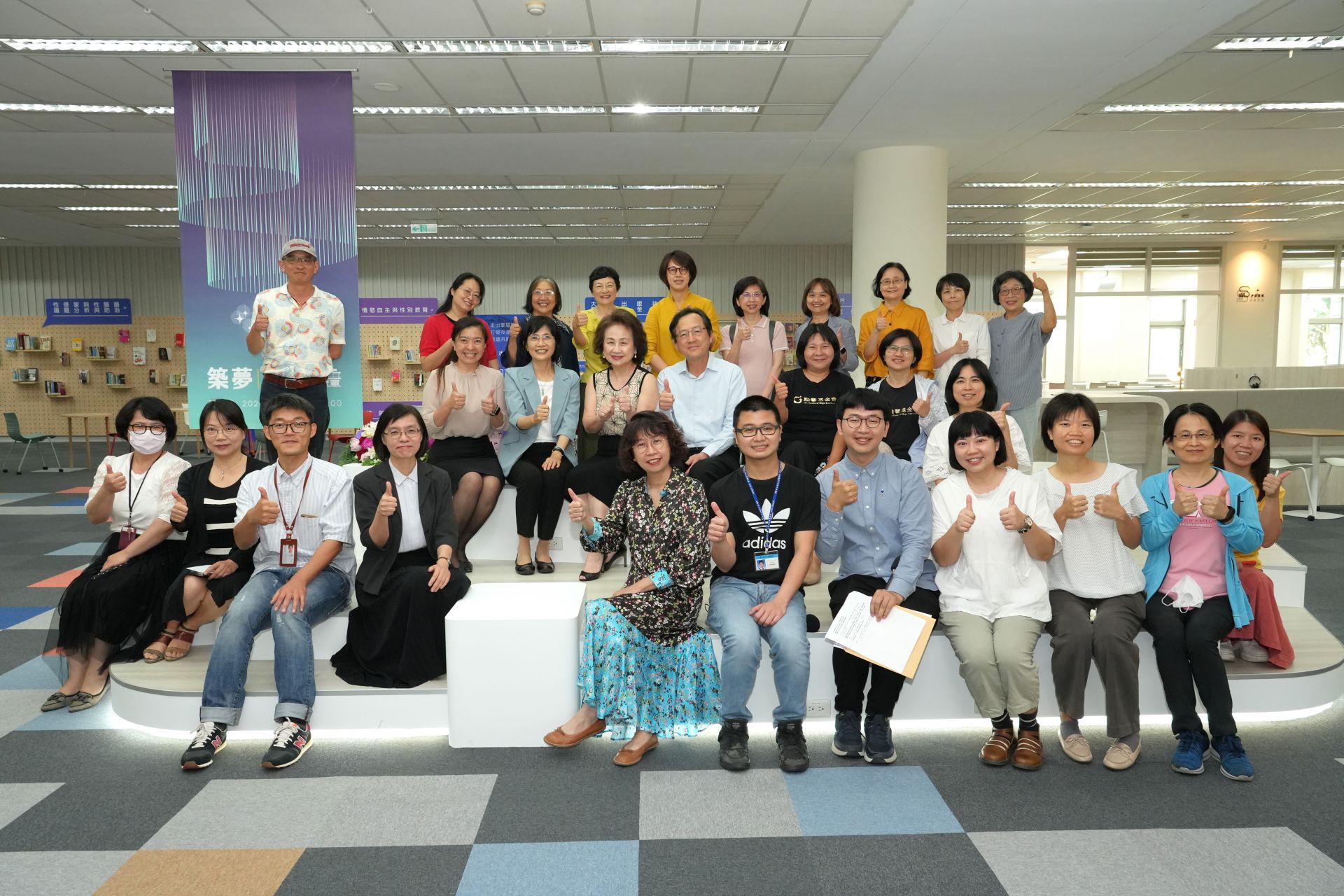
SDG11Dream into Reality, Building Dreams Solidly: Completion and Inauguration of Phase Two Space Transformation at NCKU Library
View more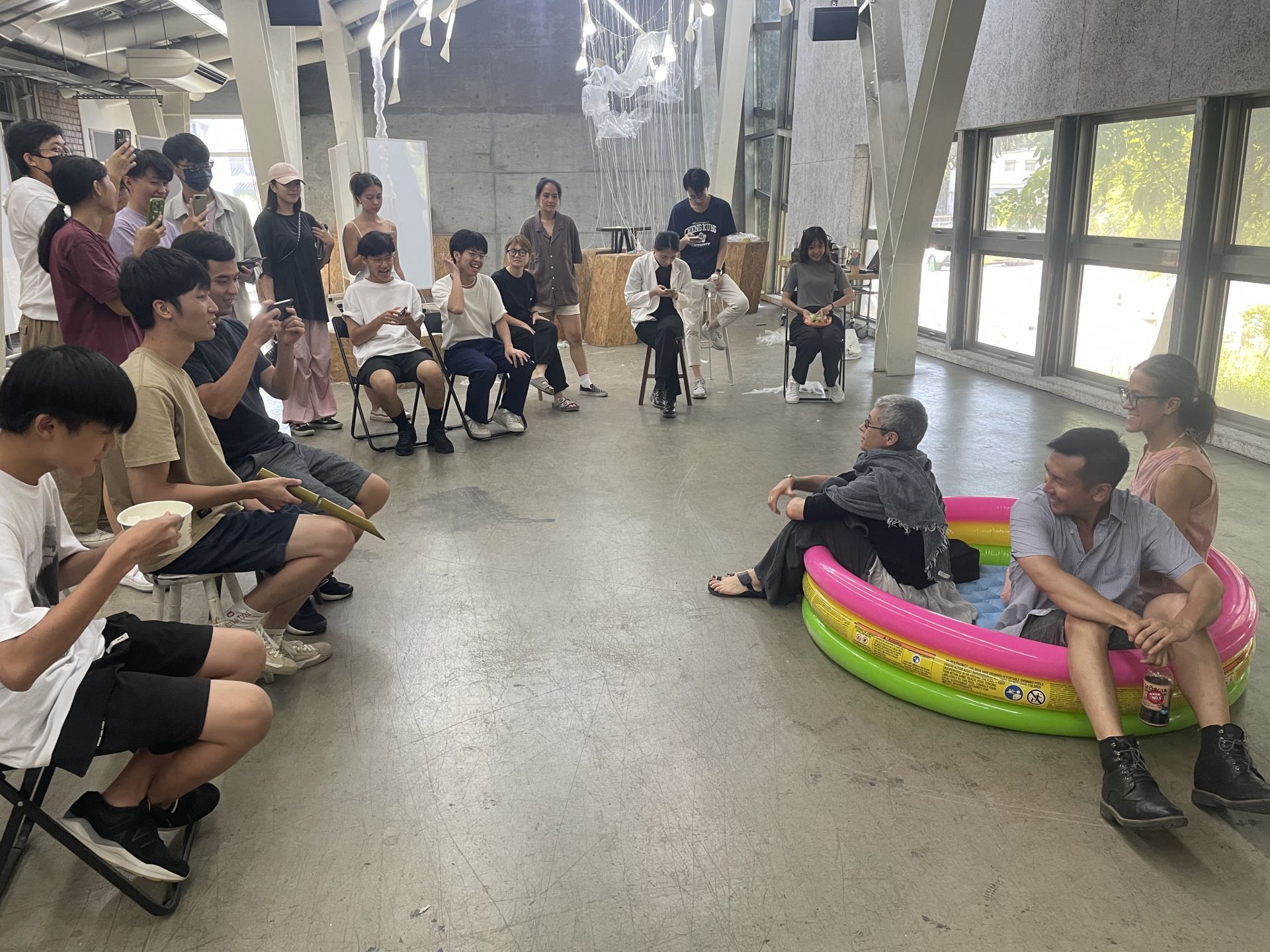